Entities
View all entitiesCSETv0 Taxonomy Classifications
Taxonomy DetailsProblem Nature
Robustness
Physical System
Software only
Level of Autonomy
Medium
Nature of End User
Amateur
Public Sector Deployment
No
Data Inputs
User posts
CSETv1 Taxonomy Classifications
Taxonomy DetailsIncident Number
84
AI Tangible Harm Level Notes
3.3 - Although there was no tangible harm, the AI was linked to the adverse outcome described in the incident.
Notes (special interest intangible harm)
AI failed to prevent the spread of misinformation
Special Interest Intangible Harm
yes
Date of Incident Year
2019
Date of Incident Month
10
Risk Subdomain
7.3. Lack of capability or robustness
Risk Domain
- AI system safety, failures, and limitations
Entity
AI
Timing
Post-deployment
Intent
Unintentional
Incident Reports
Reports Timeline
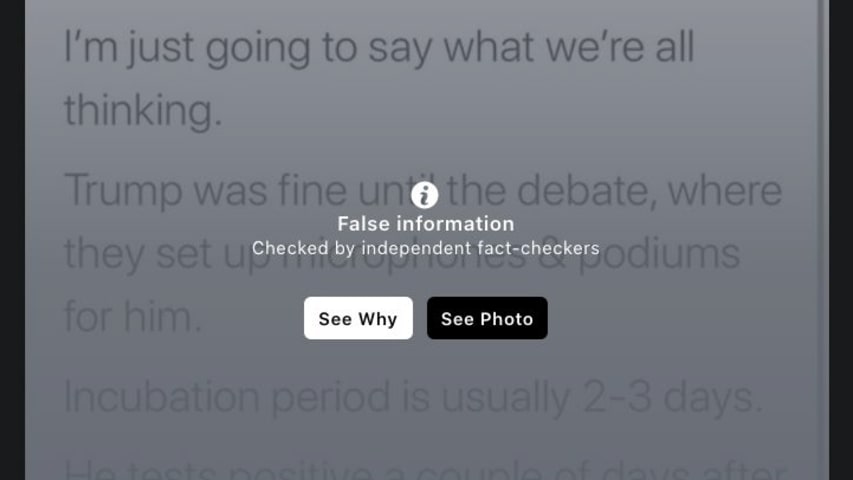
Something as simple as changing the font of a message or cropping an image can be all it takes to bypass Facebook's defenses against hoaxes and lies.
A new analysis by the international advocacy group Avaaz shines light on why, despite the …
Variants
Similar Incidents
Did our AI mess up? Flag the unrelated incidents
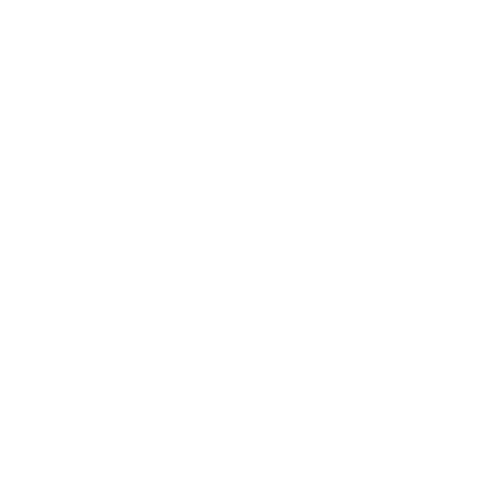
Wikipedia Vandalism Prevention Bot Loop
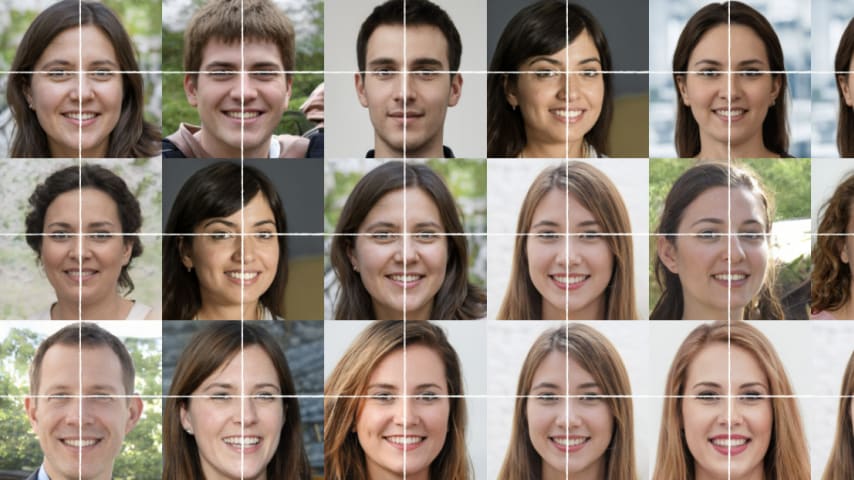
Fake LinkedIn Profiles Created Using GAN Photos
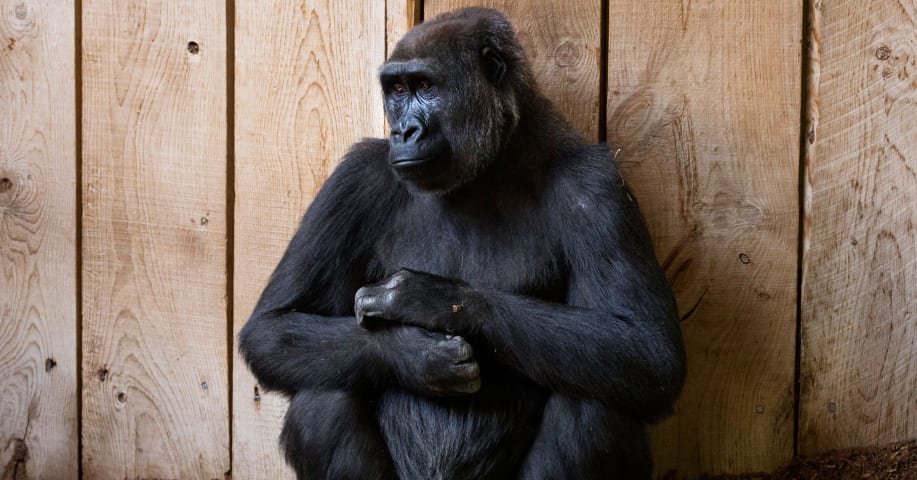
Images of Black People Labeled as Gorillas
Similar Incidents
Did our AI mess up? Flag the unrelated incidents
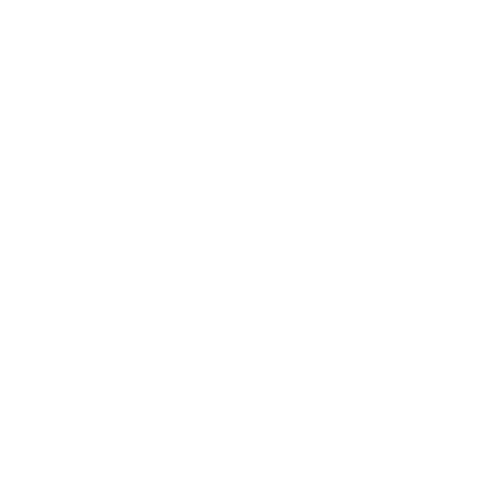
Wikipedia Vandalism Prevention Bot Loop
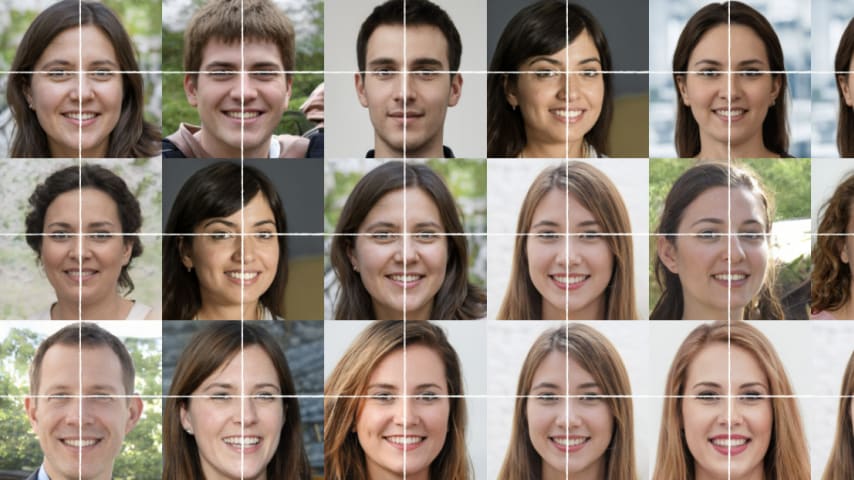
Fake LinkedIn Profiles Created Using GAN Photos
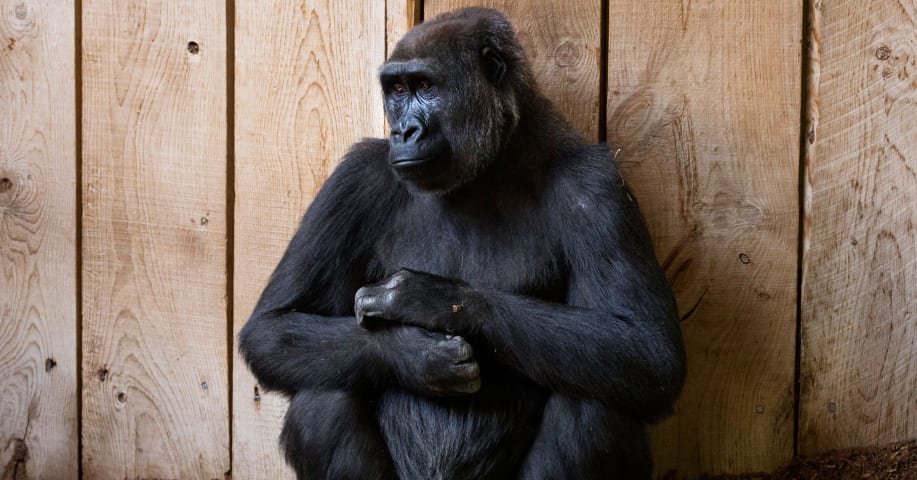