Entities
View all entitiesIncident Stats
CSETv1 Taxonomy Classifications
Taxonomy DetailsHarm Distribution Basis
financial means, race
Sector of Deployment
law enforcement
CSETv1_Annotator-1 Taxonomy Classifications
Taxonomy DetailsHarm Distribution Basis
financial means, race
Sector of Deployment
law enforcement
CSETv1_Annotator-2 Taxonomy Classifications
Taxonomy DetailsHarm Distribution Basis
race, financial means
Sector of Deployment
law enforcement
CSETv0 Taxonomy Classifications
Taxonomy DetailsFull Description
Predictive policing algorithms meant to aid law enforcement by predicting future crime show signs of biased output. PredPol, used by the Oakland (California) Police Department, and the Strategic Subject List, used by Chicago PD, were subjects of studies in 2015 and 2016 showing their bias against "low-income, minority neighborhoods." These neighborhoods would receive added attention from police departments expecting crimes to be more prevalent in the area. Notably, Oakland Police Department used 2010's record of drug crime as their baseline to train the system.
Short Description
Predictive policing algorithms meant to aid law enforcement by predicting future crime show signs of biased output.
Severity
Minor
Harm Distribution Basis
Race, National origin or immigrant status, Financial means
Harm Type
Harm to civil liberties
AI System Description
Predictive policing algorithms meant to aid police in predicting future crime.
System Developer
PredPol, Chicago Police Department
Sector of Deployment
Public administration and defence
Relevant AI functions
Cognition
AI Techniques
machine learning
AI Applications
Predictive policing
Named Entities
Oakland Police Department, Chicago Police Department, PredPol, Human Rights Data Analysis Group, Strategic Subject List
Technology Purveyor
PredPol, Chicago Police Department, Oakland Police Department
Beginning Date
2015-01-01T00:00:00.000Z
Ending Date
2017-01-01T00:00:00.000Z
Near Miss
Unclear/unknown
Intent
Unclear
Lives Lost
No
Laws Implicated
Fourth Amendment of the US Constitution
Data Inputs
Crime statistics
Incident Reports
Reports Timeline
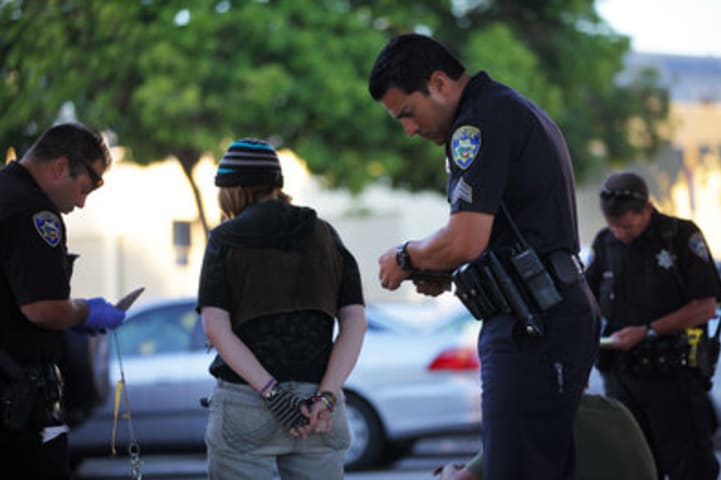
- View the original report at its source
- View the report at the Internet Archive
Faiza Patel is the co-director of the Liberty and National Security Program at the Brennan Center for Justice at New York University Law School. She is on Twitter.
In every age, police forces gain access to new tools that may advance their …
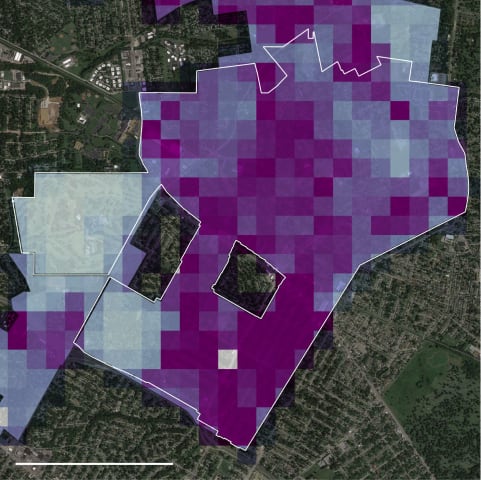
- View the original report at its source
- View the report at the Internet Archive
Just over a year after Michael Brown’s death became a focal point for a national debate about policing and race, Ferguson and nearby St. Louis suburbs have returned to what looks, from the outside, like a kind of normalcy. Near the Canfield…
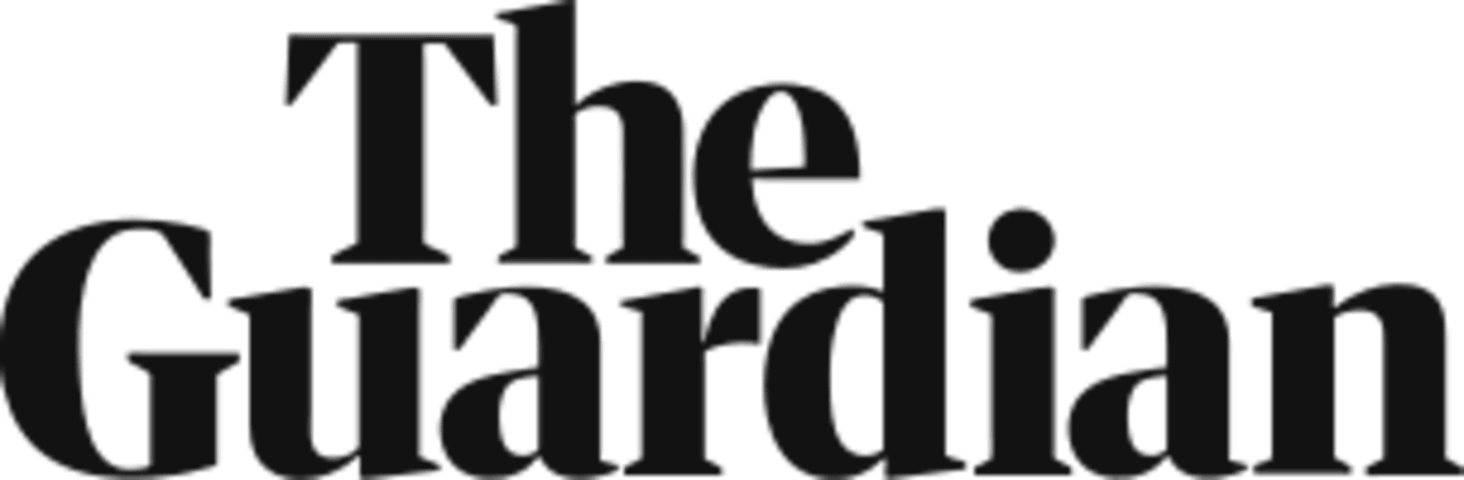
- View the original report at its source
- View the report at the Internet Archive
A police officer stands at the corner of a busy intersection, scanning the crowd with her body camera. The feed is live-streamed into the Real Time Crime Center at department headquarters, where specialized software uses biometric recogniti…
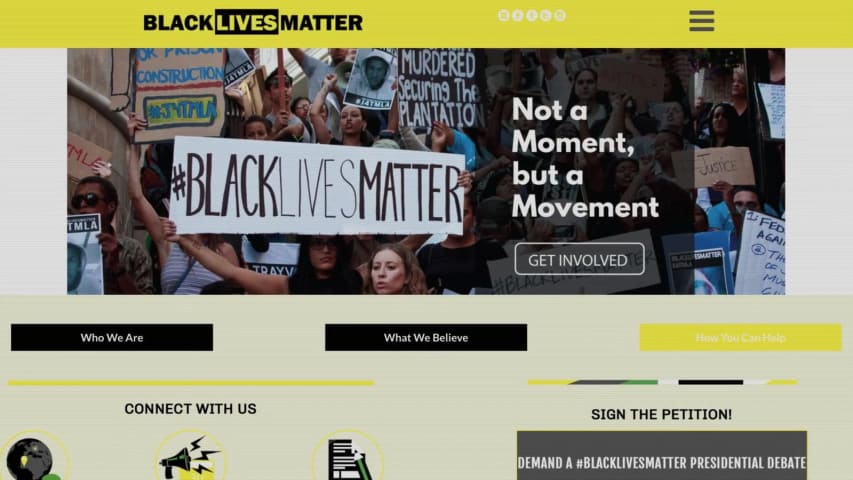
- View the original report at its source
- View the report at the Internet Archive
This is Episode 12 of Real Future, Fusion’s documentary series about technology and society. More episodes available at realfuture.tv.
There's a new kind of software that claims to help law enforcement agencies reduce crime, by using algori…
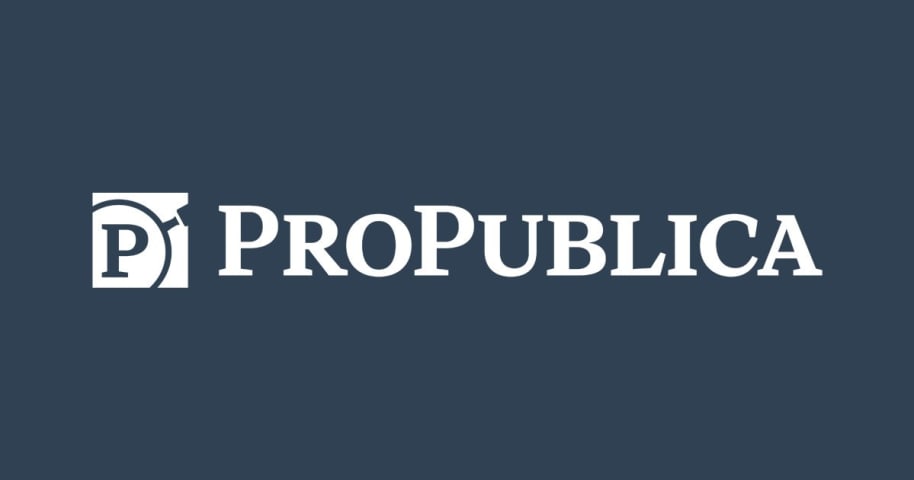
- View the original report at its source
- View the report at the Internet Archive
ON A SPRING AFTERNOON IN 2014, Brisha Borden was running late to pick up her god-sister from school when she spotted an unlocked kid’s blue Huffy bicycle and a silver Razor scooter. Borden and a friend grabbed the bike and scooter and tried…
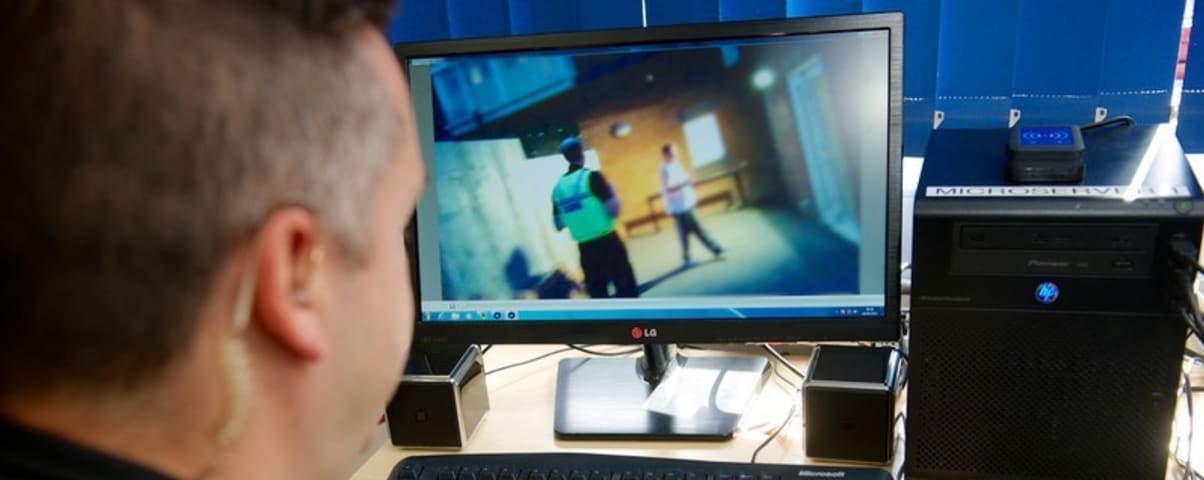
- View the original report at its source
- View the report at the Internet Archive
“Predictive policing” is happening now — and police could learn a lesson from Minority Report.
David Robinson Blocked Unblock Follow Following Aug 31, 2016
In the movie Minority Report, mutants in a vat look into the future, and tell Tom Cr…
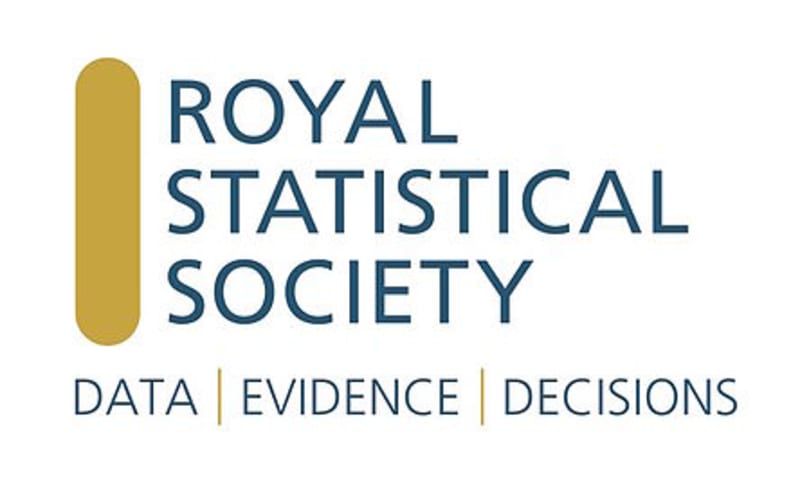
- View the original report at its source
- View the report at the Internet Archive
In late 2013, Robert McDaniel – a 22-year-old black man who lives on the South Side of Chicago – received an unannounced visit by a Chicago Police Department commander to warn him not to commit any further crimes. The visit took McDaniel by…
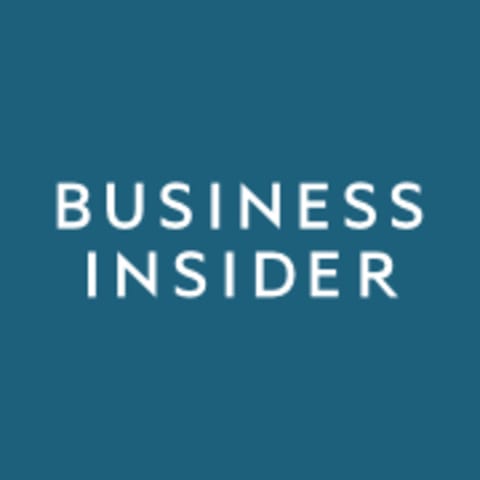
- View the original report at its source
- View the report at the Internet Archive
Natalie Behring/Getty
Algorithms have taken hold over our lives whether we appreciate it or not.
When Facebook delivers us clickbait and conspiracy theories, it's an algorithm deciding what you're interested in.
When Uber ratchets up rush-h…
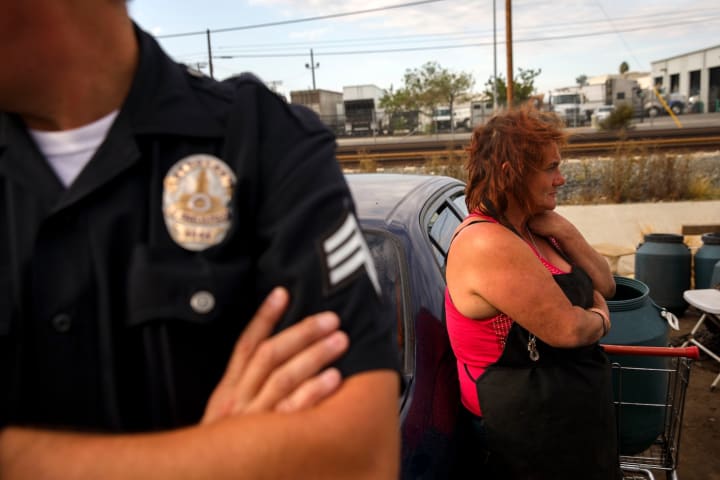
- View the original report at its source
- View the report at the Internet Archive
During an October shift, Los Angeles police Sgt. Charles Coleman of the Foothill Division speaks with Clarance Dolberry, wearing baseball cap, and Veronica De Leon, donning a Mardi Gras mask, at a bus stop. Software that predicts possible f…
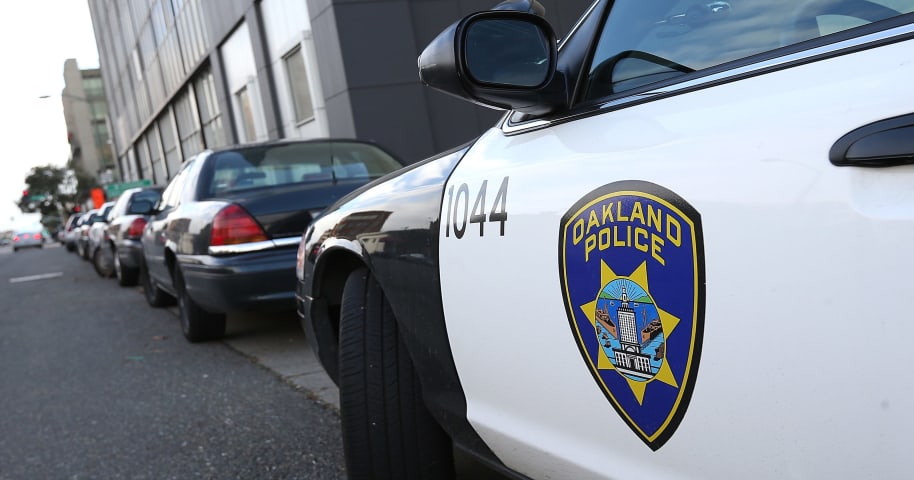
- View the original report at its source
- View the report at the Internet Archive
From Los Angeles to New York, there is a quiet revolution underway within police departments across the country.
Just as major tech companies and political campaigns have leveraged data to target potential customers or voters, police depart…
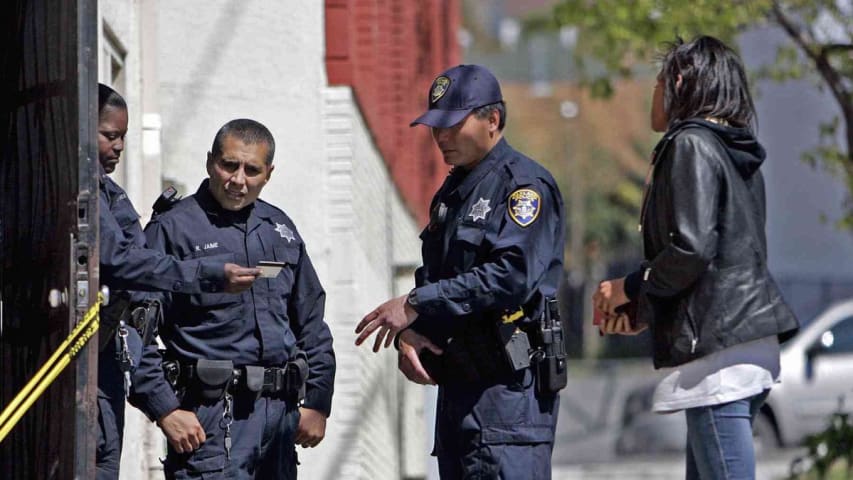
- View the original report at its source
- View the report at the Internet Archive
Image: Gina Ferazzi/Getty
Tim Birch was six months into his new job as head of research and planning for the Oakland Police Department when he walked into his office and found a piece of easel pad paper tacked onto his wall. Scribbled acros…
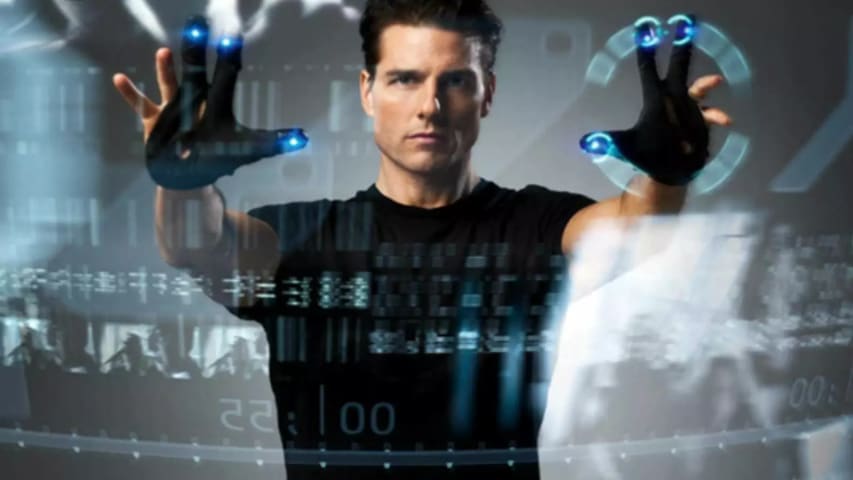
- View the original report at its source
- View the report at the Internet Archive
The problem of policing has always been that it's after-the-fact. If law enforcement officers could be at the right place at the right time, crime could be prevented, lives could be saved, and society would surely be safer. In recent years,…
- View the original report at its source
- View the report at the Internet Archive
The Truth About Predictive Policing and Race
Sunday, the New York Times published a well-meaning op-ed about the fears of racial bias in artificial intelligence and predictive policing systems. The author, Bärí A. Williams, should be commen…
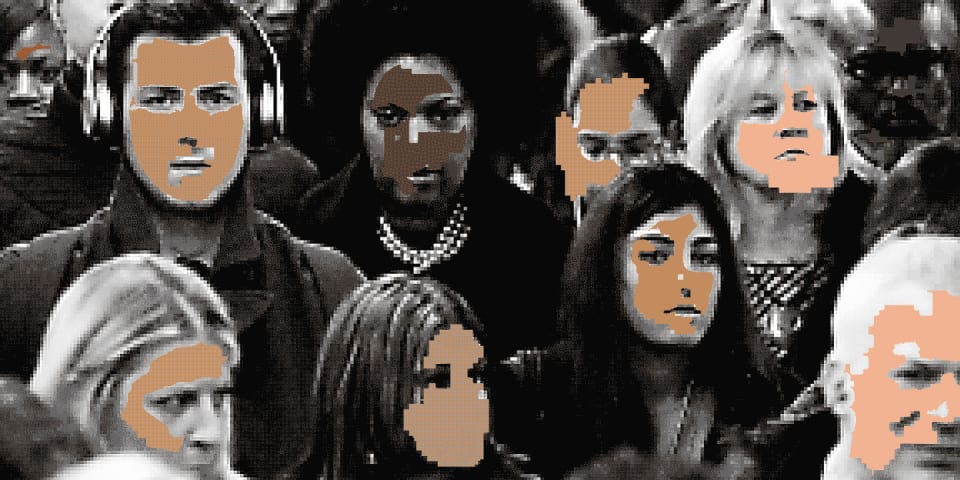
- View the original report at its source
- View the report at the Internet Archive
IN THE DECADE after the 9/11 attacks, the New York City Police Department moved to put millions of New Yorkers under constant watch. Warning of terrorism threats, the department created a plan to carpet Manhattan’s downtown streets with tho…
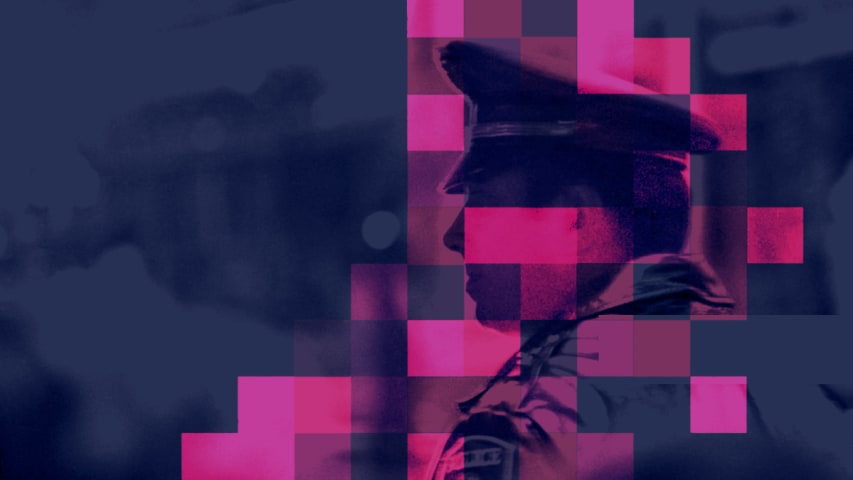
- View the original report at its source
- View the report at the Internet Archive
Introduction
The expansion of digital record-keeping by police departments across the U.S. in the 1990s ushered in the era of data-driven policing. Huge metropolises like New York City crunched reams of crime and arrest data to find and tar…
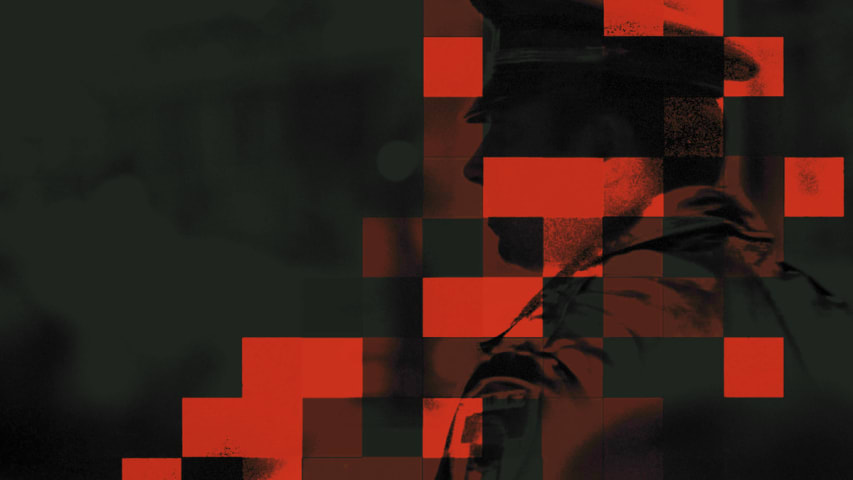
- View the original report at its source
- View the report at the Internet Archive
Between 2018 and 2021, more than one in 33 U.S. residents were potentially subject to police patrol decisions directed by crime-prediction software called PredPol.
The company that makes it sent more than 5.9 million of these crime predicti…
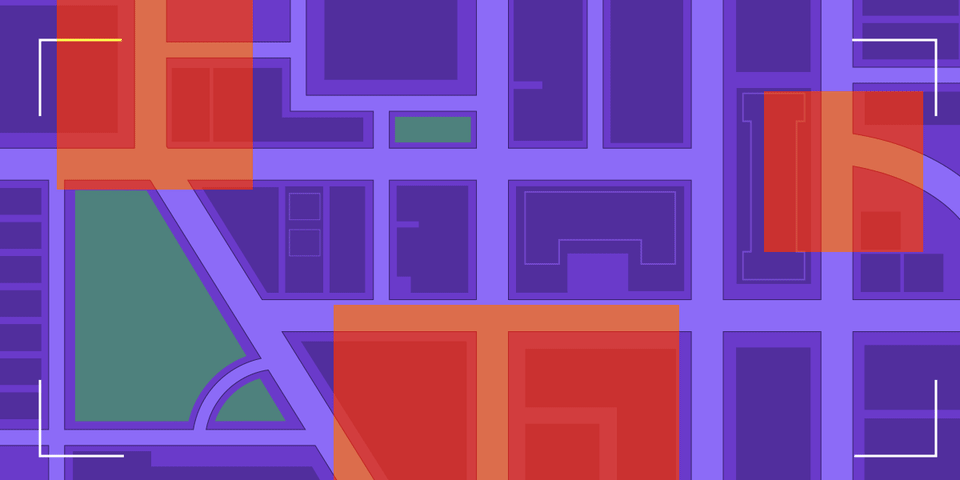
- View the original report at its source
- View the report at the Internet Archive
Decades ago, when imagining the practical uses of artificial intelligence, science fiction writers imagined autonomous digital minds that could serve humanity. Sure, sometimes a HAL 9000 or WOPR would subvert expectations and go rogue, but …
Variants
Similar Incidents
Did our AI mess up? Flag the unrelated incidents
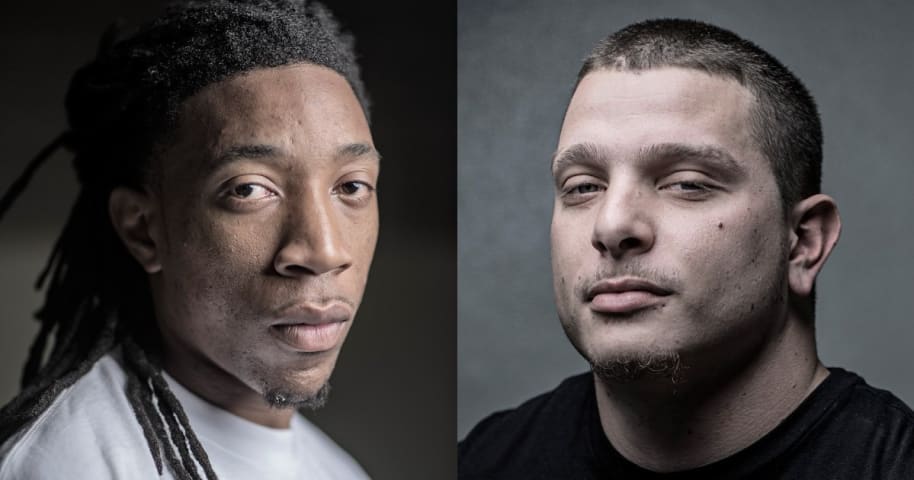
Northpointe Risk Models
Sexist and Racist Google Adsense Advertisements
Similar Incidents
Did our AI mess up? Flag the unrelated incidents
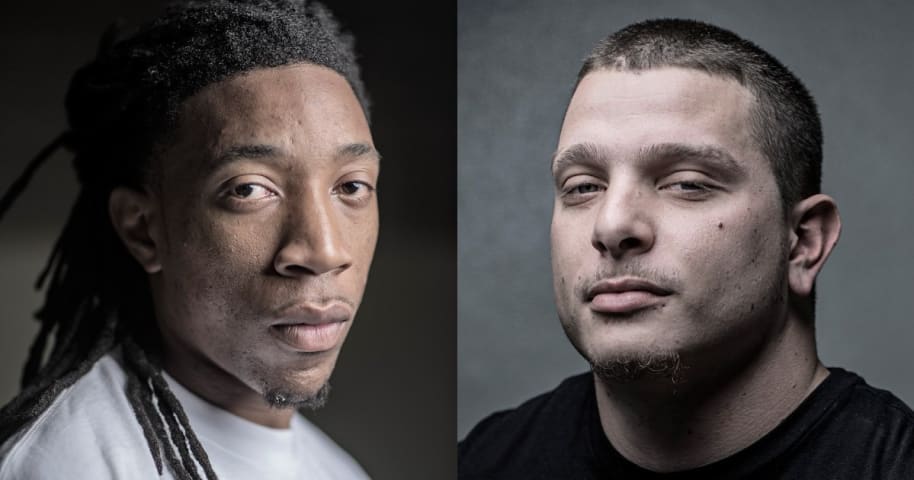