Entities
View all entitiesIncident Stats
CSETv0 Taxonomy Classifications
Taxonomy DetailsProblem Nature
Unknown/unclear
Physical System
Software only
Level of Autonomy
Medium
Nature of End User
Expert
Public Sector Deployment
Yes
Data Inputs
137-question survey
CSETv1 Taxonomy Classifications
Taxonomy DetailsIncident Number
11
CSETv1_Annotator-1 Taxonomy Classifications
Taxonomy DetailsIncident Number
11
CSETv1_Annotator-2 Taxonomy Classifications
Taxonomy DetailsIncident Number
11
AI Tangible Harm Level Notes
A clear disparity between the assessment of white individuals and POC individuals.
Notes (special interest intangible harm)
Algorithm incorporates race in determining the likelihood for individuals to commit more crimes after sentencing. As a result, black individuals receive harsher sentences than white counterparts.
Special Interest Intangible Harm
yes
Incident Reports
Reports Timeline
- View the original report at its source
- View the report at the Internet Archive
Computer programs that perform risk assessments of crime suspects are increasingly common in American courtrooms, and are used at every stage of the criminal justice systems to determine who may be set free or granted parole, and the size o…
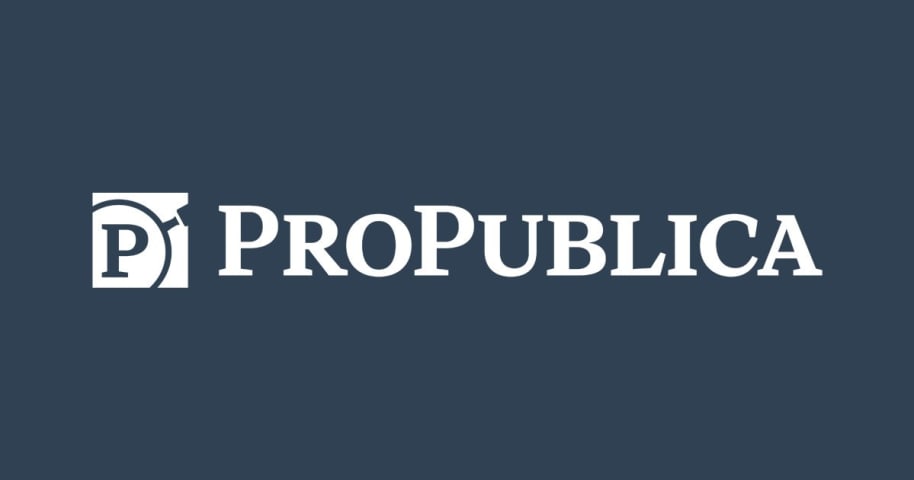
- View the original report at its source
- View the report at the Internet Archive
Across the nation, judges, probation and parole officers are increasingly using algorithms to assess a criminal defendant’s likelihood of becoming a recidivist – a term used to describe criminals who re-offend. There are dozens of these ris…

- View the original report at its source
- View the report at the Internet Archive
For years, the criminal justice community has been worried. Courts across the country are assigning bond amounts sentencing the accused based on algorithms, and both lawyers and data scientists warn that these algorithms could be poisoned b…
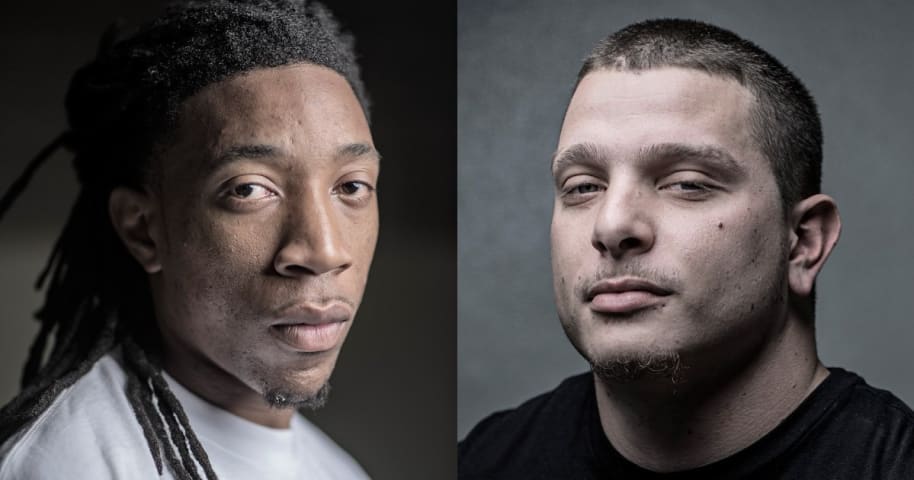
- View the original report at its source
- View the report at the Internet Archive
On a spring afternoon in 2014, Brisha Borden was running late to pick up her god-sister from school when she spotted an unlocked kid’s blue Huffy bicycle and a silver Razor scooter. Borden and a friend grabbed the bike and scooter and tried…
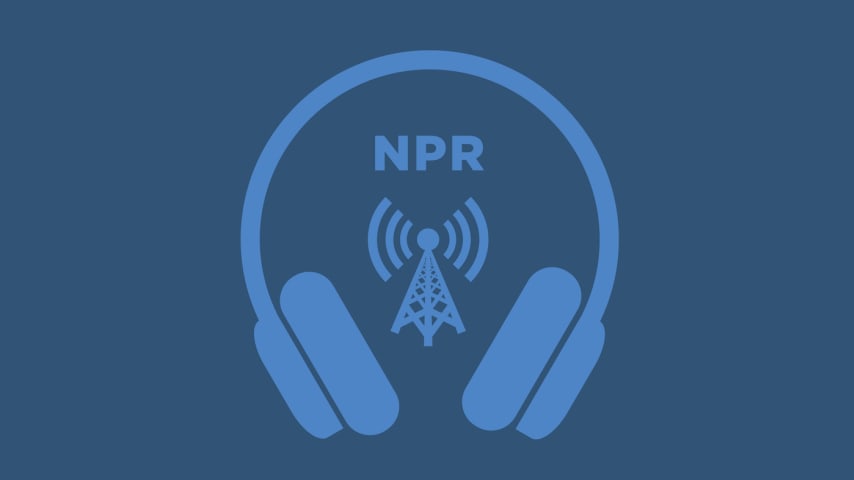
- View the original report at its source
- View the report at the Internet Archive
The Hidden Discrimination In Criminal Risk-Assessment Scores
Courtrooms across the country are increasingly using a defendant's "risk assessment score" to help make decisions about bond, parole and sentencing. The companies behind these sco…
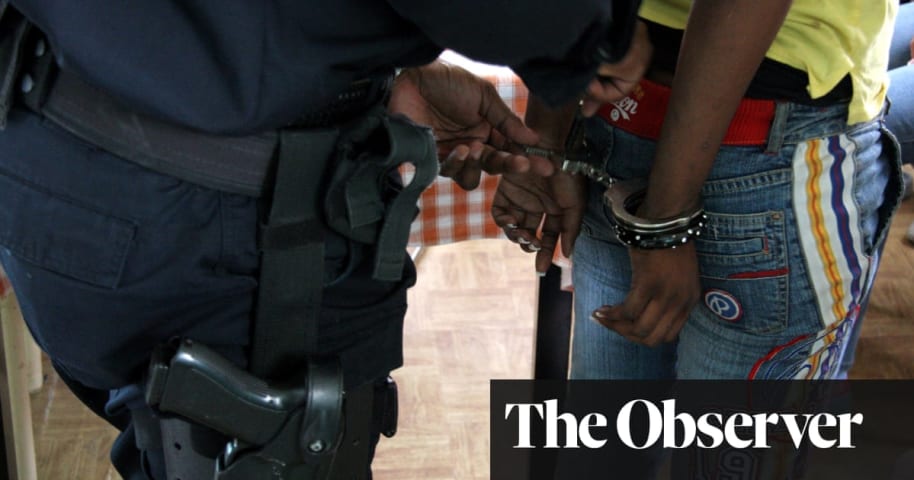
- View the original report at its source
- View the report at the Internet Archive
One of my most treasured possessions is The Art of Computer Programming by Donald Knuth, a computer scientist for whom the word “legendary” might have been coined. In a way, one could think of his magnum opus as an attempt to do for compute…
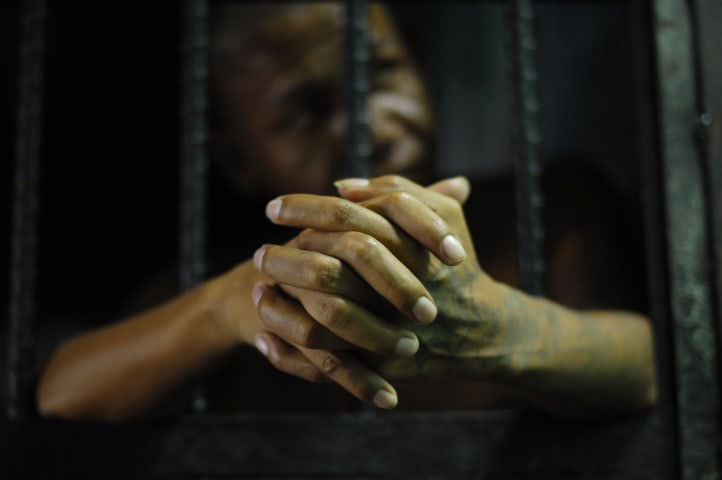
- View the original report at its source
- View the report at the Internet Archive
Imagine you were found guilty of a crime and were waiting to learn your sentence. Would you rather have your sentence determined by a computer algorithm, which dispassionately weights factors that predict your future risk of crime (such as …
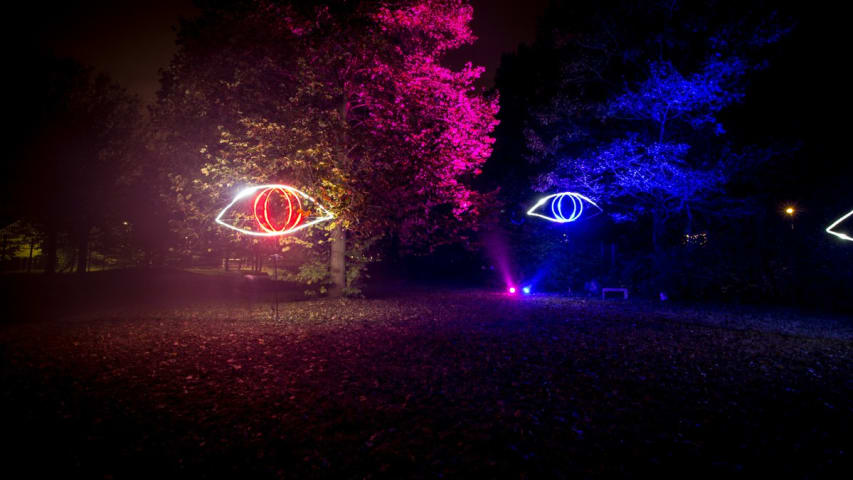
- View the original report at its source
- View the report at the Internet Archive
Like a more crooked version of the Voight-Kampff test from Blade Runner, a new machine learning paper from a pair of Chinese researchers has delved into the controversial task of letting a computer decide on your innocence. Can a computer k…
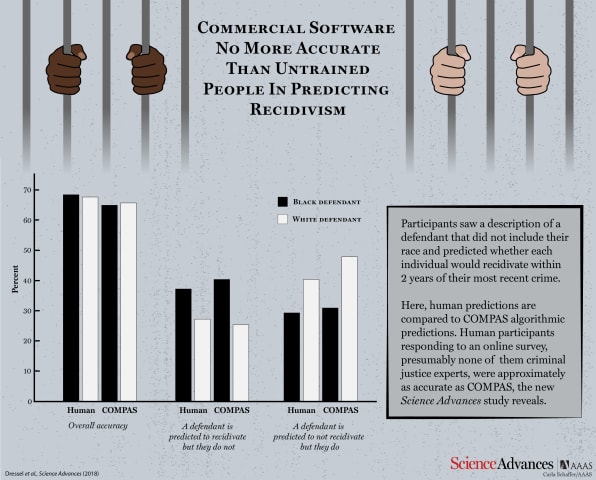
- View the original report at its source
- View the report at the Internet Archive
Predicting the future is not only the provenance of fortune tellers or media pundits. Predictive algorithms, based on extensive datasets and statistics have overtaken wholesale and retail operations as any online shopper knows. And in the l…
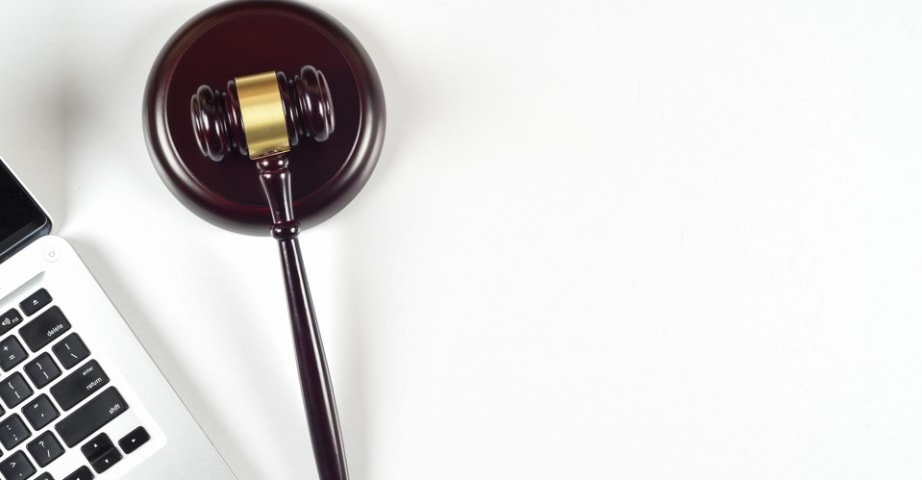
- View the original report at its source
- View the report at the Internet Archive
Caution is indeed warranted, according to Julia Dressel and Hany Farid from Dartmouth College. In a new study, they have shown that COMPAS is no better at predicting an individual’s risk of recidivism than random volunteers recruited from t…
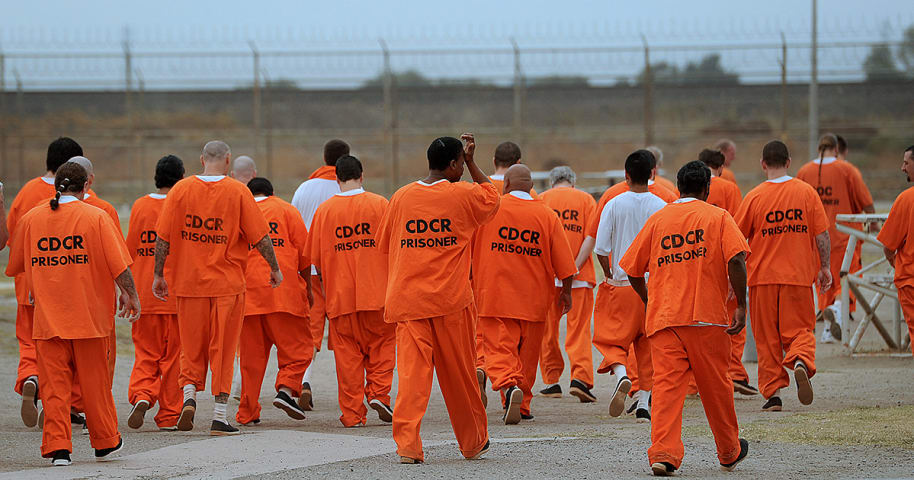
- View the original report at its source
- View the report at the Internet Archive
Don’t blame the algorithm — as long as there are racial disparities in the justice system, sentencing software can never be entirely fair.
For generations, the Maasai people of eastern Africa have passed down the story of a tireless old man…
- View the original report at its source
- View the report at the Internet Archive
Although crime rates have fallen steadily since the 1990s, rates of recidivism remain a factor in the areas of both public safety and prisoner management. The National Institute of Justice defines recidivism as “criminal acts that resulted …
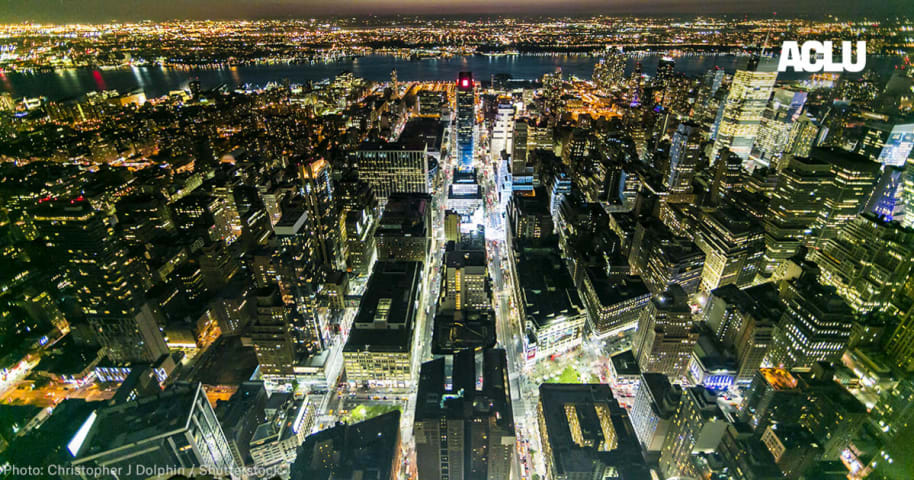
- View the original report at its source
- View the report at the Internet Archive
Invisible algorithms increasingly shape the world we live in, and not always for the better. Unfortunately, few mechanisms are in place to ensure they’re not causing more harm than good.
That might finally be changing: A first-in-the-nation…
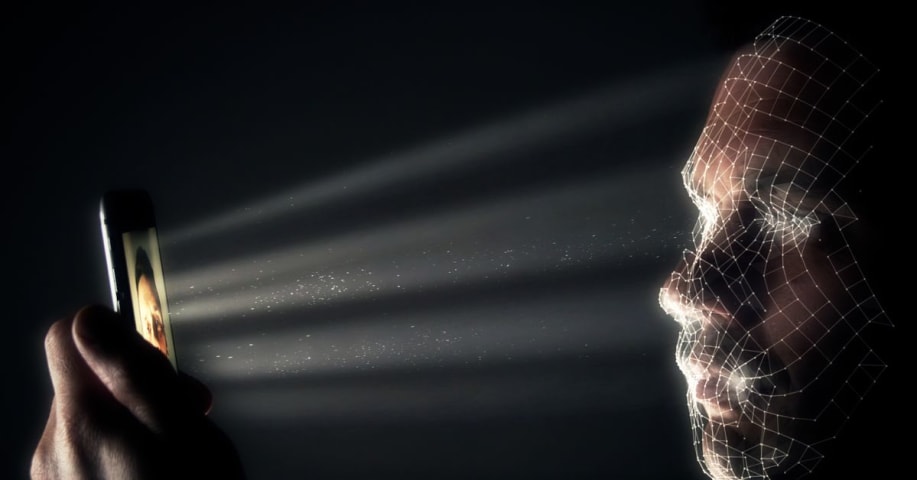
- View the original report at its source
- View the report at the Internet Archive
Open up the photo app on your phone and search “dog,” and all the pictures you have of dogs will come up. This was no easy feat. Your phone knows what a dog “looks” like.
This modern-day marvel is the result of machine learning, a form of a…
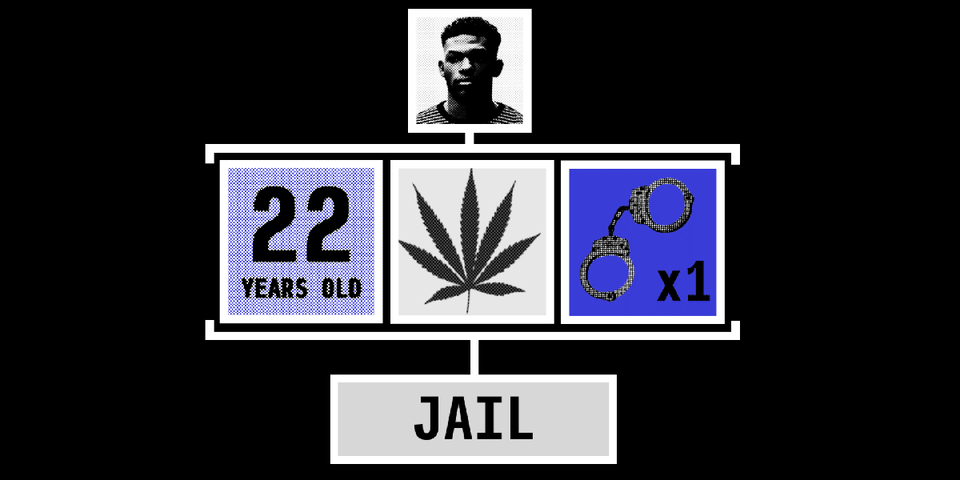
- View the original report at its source
- View the report at the Internet Archive
As a child, you develop a sense of what “fairness” means. It’s a concept that you learn early on as you come to terms with the world around you. Something either feels fair or it doesn’t.
But increasingly, algorithms have begun to arbitrate…
Variants
Similar Incidents
Did our AI mess up? Flag the unrelated incidents
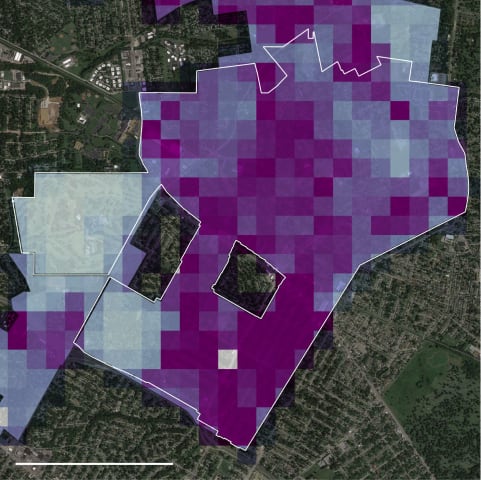
Predictive Policing Biases of PredPol
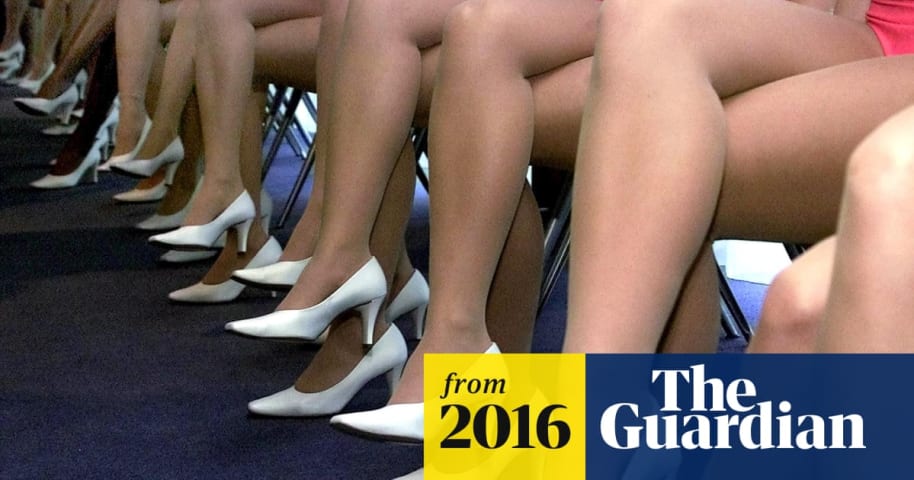
AI Beauty Judge Did Not Like Dark Skin
Similar Incidents
Did our AI mess up? Flag the unrelated incidents
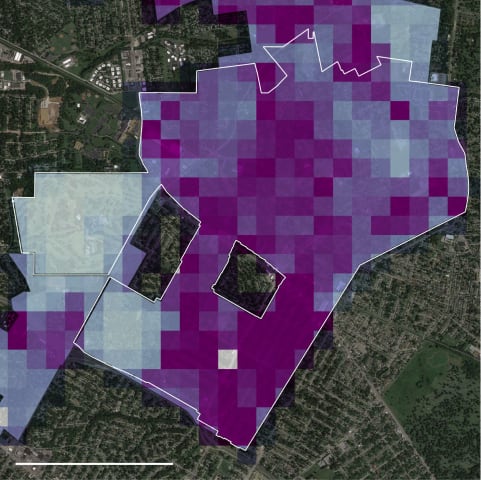
Predictive Policing Biases of PredPol
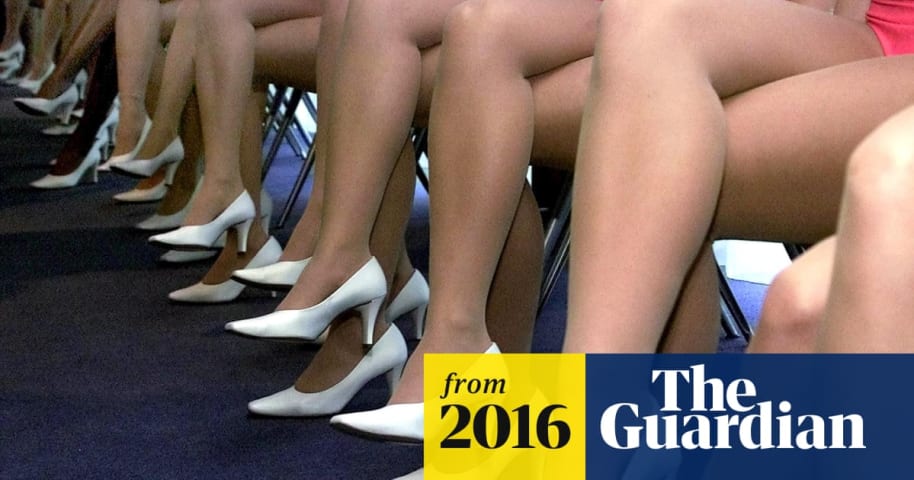