Entities
View all entitiesCSETv0 Taxonomy Classifications
Taxonomy DetailsProblem Nature
Unknown/unclear
Physical System
Software only
Level of Autonomy
Medium
Nature of End User
Amateur
Public Sector Deployment
Yes
Data Inputs
Questionnaire consisting of 137 factors like age, prior convictions, criminal records
CSETv1 Taxonomy Classifications
Taxonomy DetailsIncident Number
40
AI Tangible Harm Level Notes
CSET considers wrongful detention, wrongful imprisonment, and wrongful differential/disproportionate imprisonment amounts to be tangible harm, because of the loss of physical freedom and autonomy.
Risk Subdomain
1.1. Unfair discrimination and misrepresentation
Risk Domain
- Discrimination and Toxicity
Entity
AI
Timing
Post-deployment
Intent
Unintentional
Incident Reports
Reports Timeline
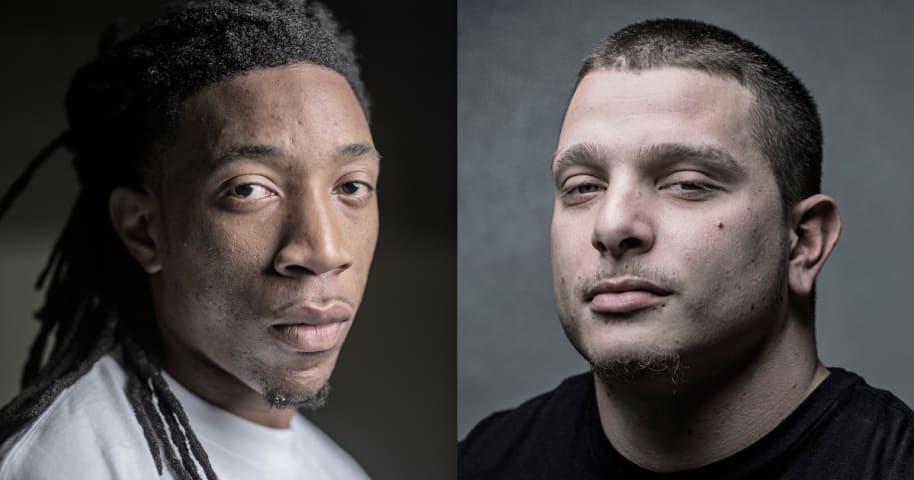
← Read the story
Across the nation, judges, probation and parole officers are increasingly using algorithms to assess a criminal defendant’s likelihood of becoming a recidivist – a term used to describe criminals who re-offend. There are do…
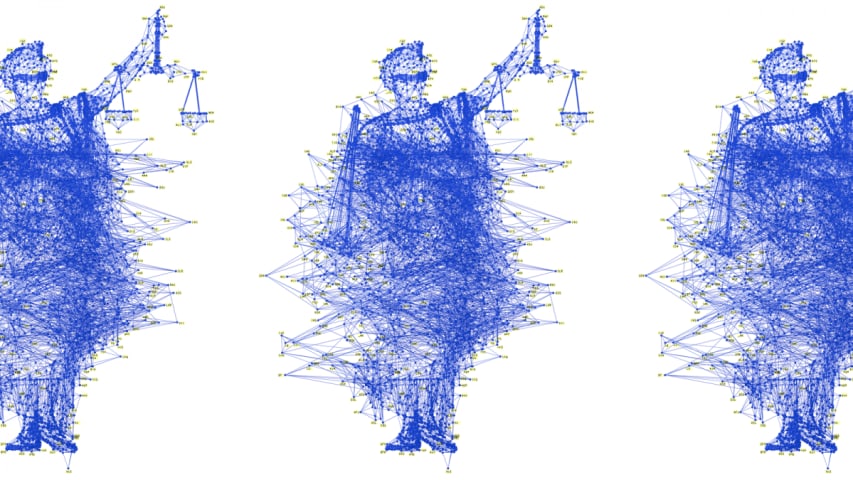
It was a striking story. “Machine Bias,” the headline read, and the teaser proclaimed: “There’s software used across the country to predict future criminals. And it’s biased against blacks.”
ProPublica, a Pulitzer Prize–winning nonprofit ne…
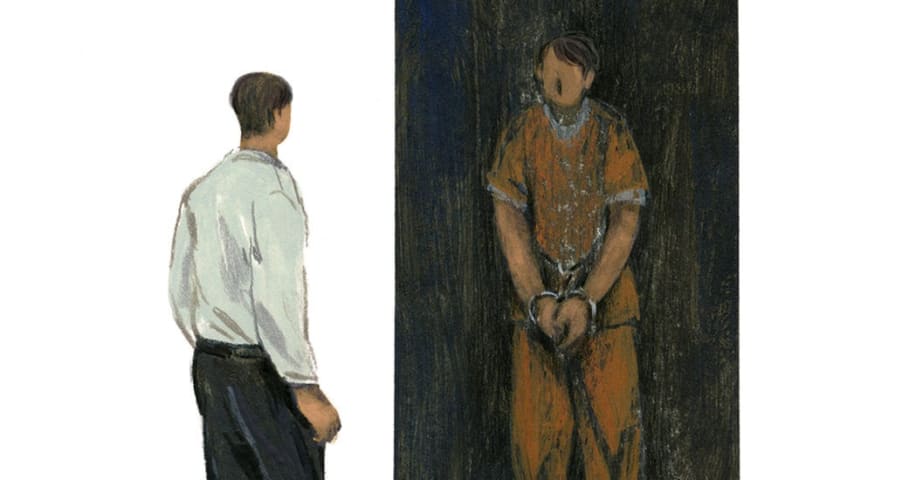
The criminal justice system is becoming automated. At every stage — from policing and investigations to bail, evidence, sentencing and parole — computer systems play a role. Artificial intelligence deploys cops on the beat. Audio sensors ge…
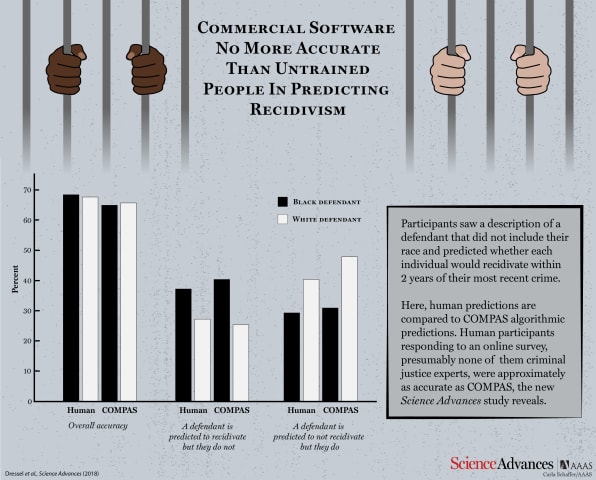
Predicting the future is not only the provenance of fortune tellers or media pundits. Predictive algorithms, based on extensive datasets and statistics have overtaken wholesale and retail operations as any online shopper knows. And in the l…
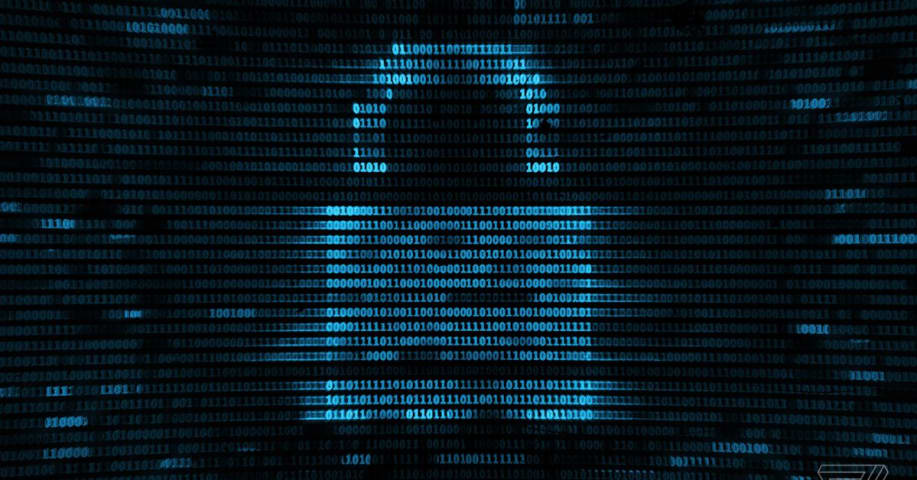
Our most sophisticated crime-predicting algorithms may not be as good as we thought. A study published today in Science Advances takes a look at the popular COMPAS algorithm — used to assess the likelihood that a given defendant will reoffe…
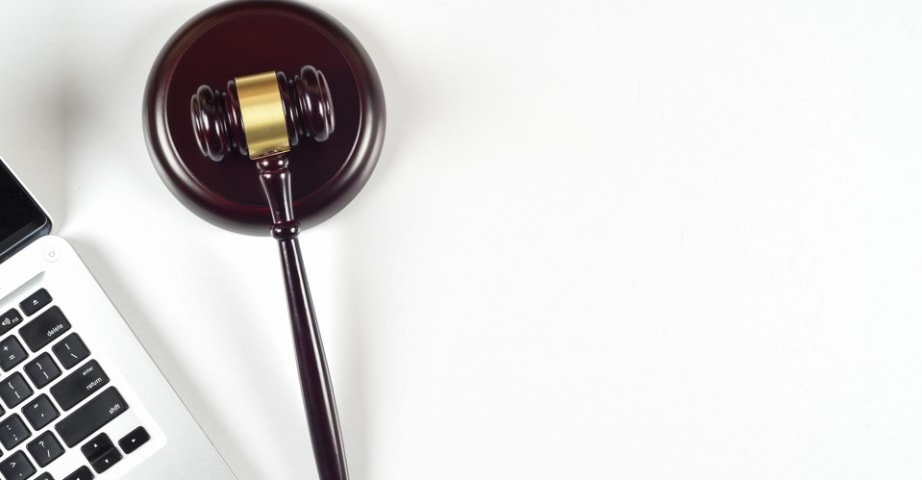
Caution is indeed warranted, according to Julia Dressel and Hany Farid from Dartmouth College. In a new study, they have shown that COMPAS is no better at predicting an individual’s risk of recidivism than random volunteers recruited from t…
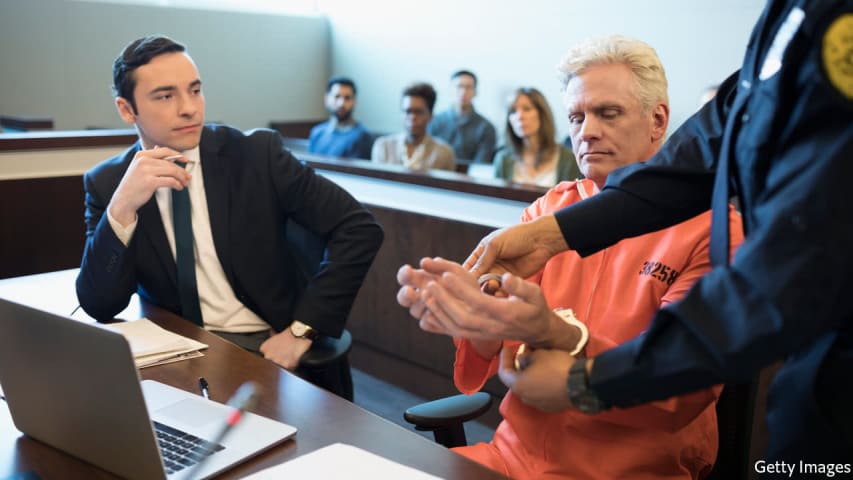
IN AMERICA, computers have been used to assist bail and sentencing decisions for many years. Their proponents argue that the rigorous logic of an algorithm, trained with a vast amount of data, can make judgments about whether a convict will…
Algorithms for predicting recidivism are commonly used to assess a criminal defendant’s likelihood of committing a crime. These predictions are used in pretrial, parole, and sentencing decisions. Proponents of these systems argue that big d…
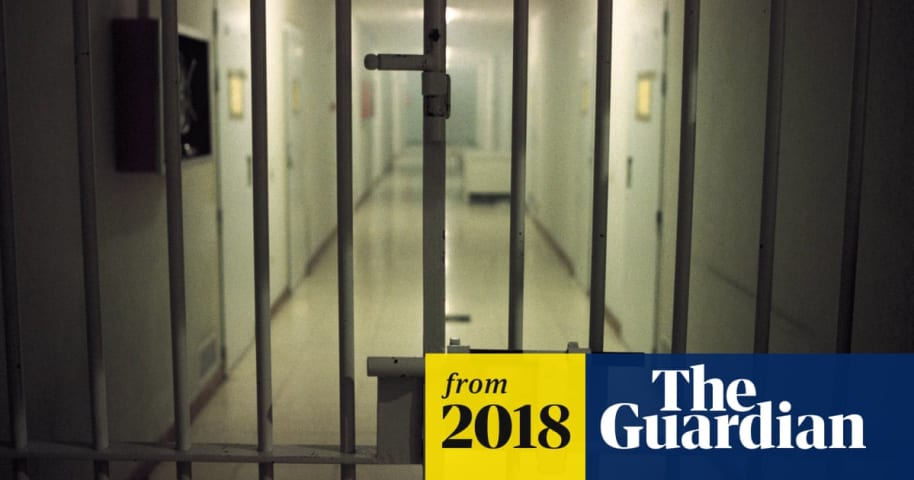
Program used to assess more than a million US defendants may not be accurate enough for potentially life-changing decisions, say experts
The credibility of a computer program used for bail and sentencing decisions has been called into quest…
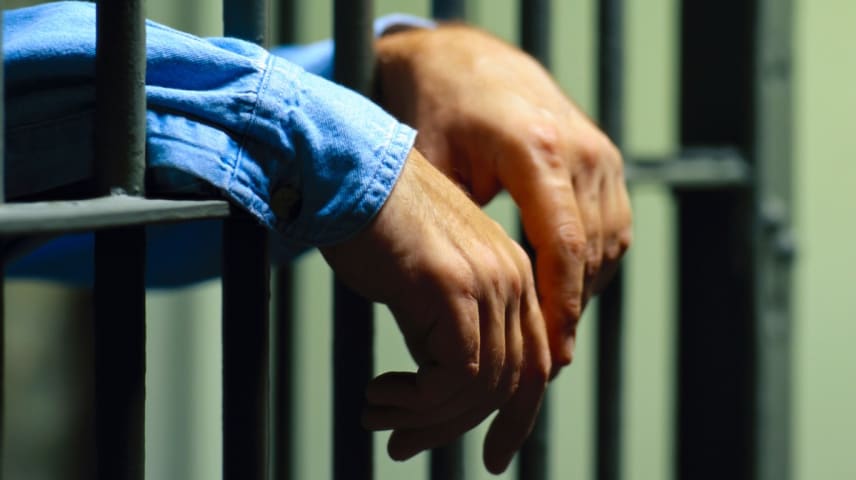
Algorithms that assess people’s likelihood to reoffend as part of the bail-setting process in criminal cases are, to be frank, really scary.
We don’t know very much about how they work—the companies that make them are intensely secretive ab…
A widely-used computer software tool may be no more accurate or fair at predicting repeat criminal behavior than people with no criminal justice experience, according to a Dartmouth College study.
The Dartmouth analysis showed that non-expe…
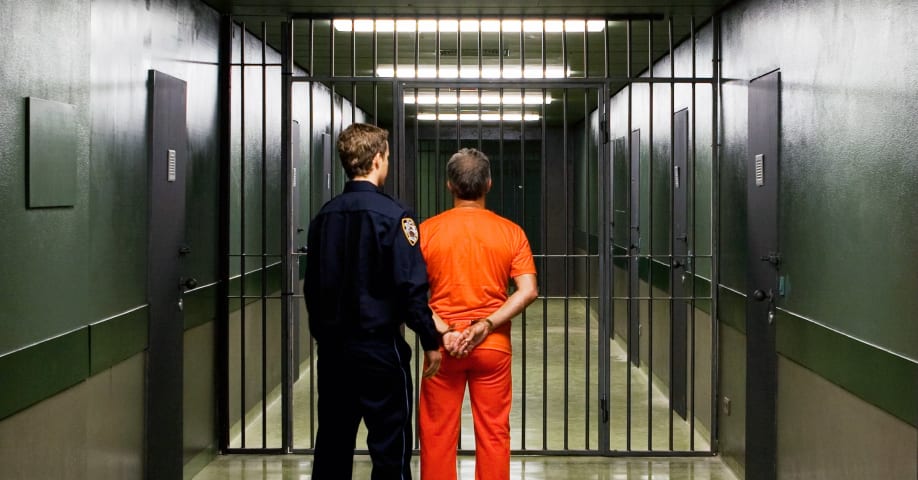
The American criminal justice system couldn’t get much less fair. Across the country, some 1.5 million people are locked up in state and federal prisons. More than 600,000 people, the vast majority of whom have yet to be convicted of a crim…
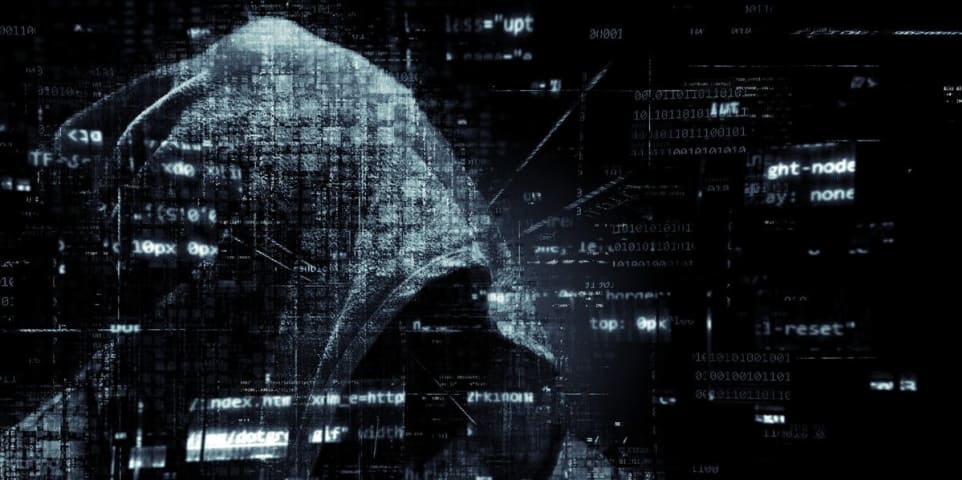
Just like a professional chef or a heart surgeon, a machine learning algorithm is only as good as the training it receives. And as algorithms increasingly take the reigns and make decisions for humans, we’re finding out that a lot of them d…

Predicting Recidivism
Recidivism is the likelihood of a person convicted of a crime to offend again. Currently, this rate is determined by predictive algorithms. The outcome can affect everything from sentencing decisions to whether or not …
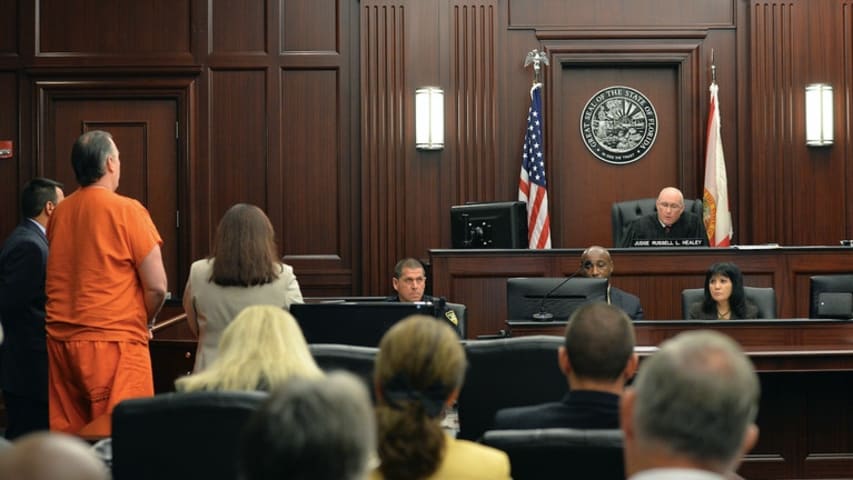
In a study published Wednesday, a pair of Dartmouth researchers found that a popular risk assessment algorithm was no better at predicting a criminal offender's likelihood of reoffending than an internet survey of humans with little or no r…

Receive emails about upcoming NOVA programs and related content, as well as featured reporting about current events through a science lens. Email Address Zip Code Subscribe
An “unbiased” computer algorithm used for informing judicial decisi…
Although crime rates have fallen steadily since the 1990s, rates of recidivism remain a factor in the areas of both public safety and prisoner management. The National Institute of Justice defines recidivism as “criminal acts that resulted …
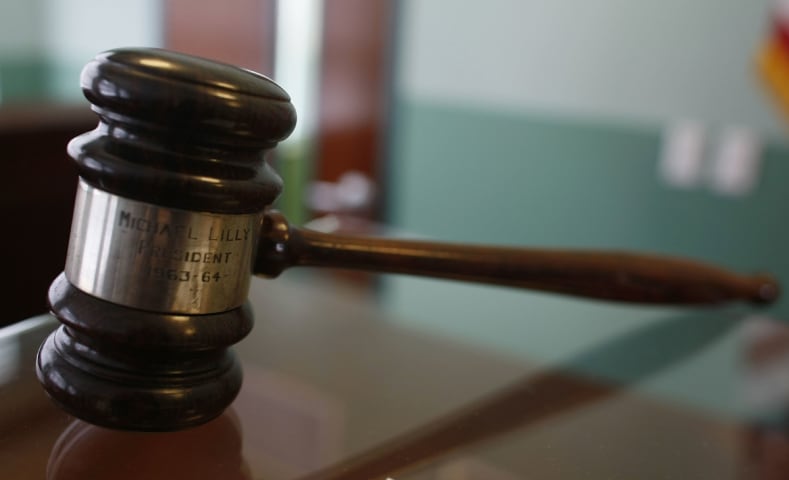
(Photo: Joe Raedle/Getty Images)
Dozens of people packed into a Philadelphia courtroom on June 6th to voice their objections to a proposed criminal justice algorithm. The algorithm, developed by the Pennsylvania Commission on Sentencing, wa…

When Netflix gets a movie recommendation wrong, you’d probably think that it’s not a big deal. Likewise, when your favourite sneakers don’t make it into Amazon’s list of recommended products, it’s probably not the end of the world. But when…
In a study of COMPAS, an algorithmic tool used in the US criminal justice system , Dartmouth College researchers Julia Dressel and Hany Farid found that the algorithm did no better than volunteers recruited via a crowdsourcing site. COMPAS,…
PRELIMINARY STATEMENT AND STATEMENT OF INTEREST
Independent and adversarial review of software used in the
criminal legal system is necessary to protect the courts from
unreliable evidence and to ensure that the introduction of new
technolo…
Recidivism risk assessment is the process of determining the likelihood that an accused, convicted, or incarcerated persons will reoffend. The process is aimed at assisting in the determination of the appropriate limitation on the freedom o…
Variants
Similar Incidents
Did our AI mess up? Flag the unrelated incidents
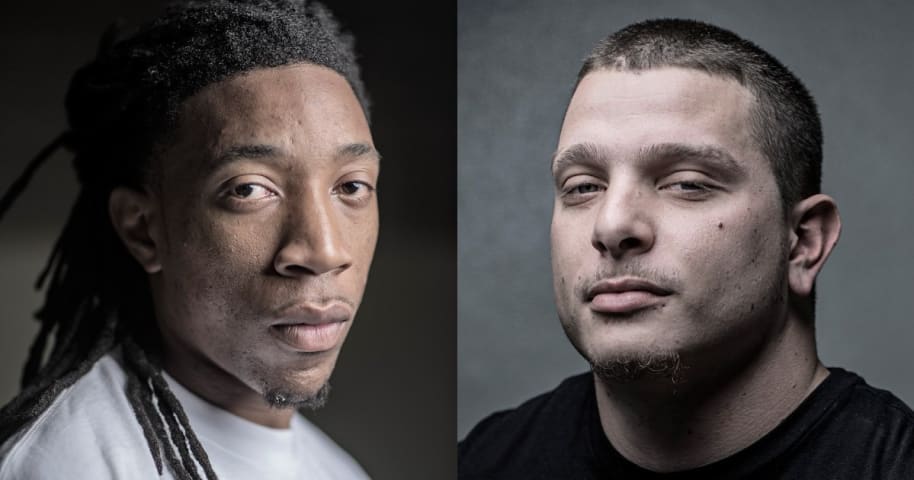
Northpointe Risk Models
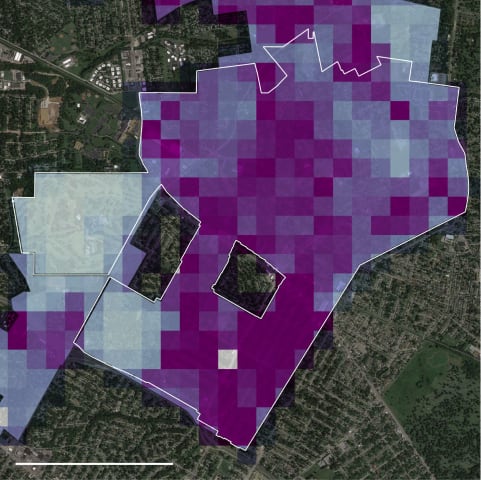
Predictive Policing Biases of PredPol
Sexist and Racist Google Adsense Advertisements
Similar Incidents
Did our AI mess up? Flag the unrelated incidents
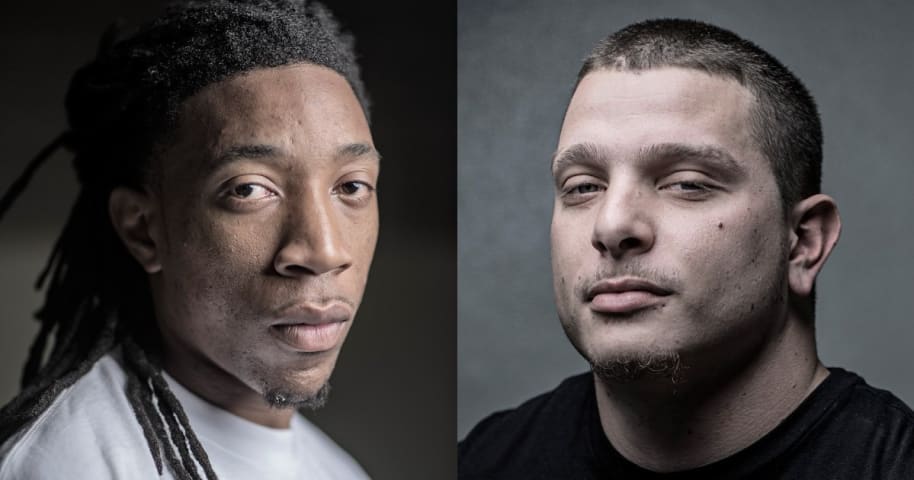
Northpointe Risk Models
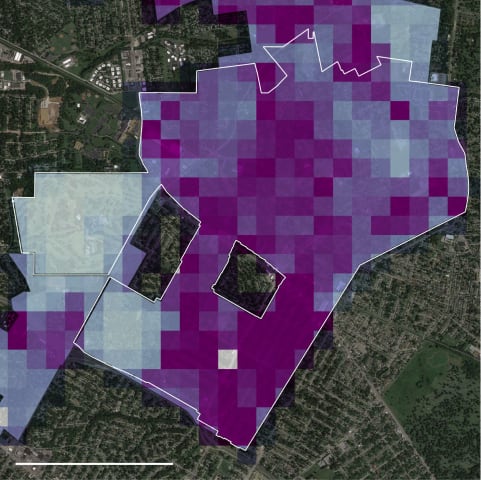