Entities
View all entitiesIncident Stats
CSETv1 Taxonomy Classifications
Taxonomy DetailsHarm Distribution Basis
race, sex, age, disability
Sector of Deployment
information and communication
CSETv1_Annotator-2 Taxonomy Classifications
Taxonomy DetailsHarm Distribution Basis
race
Sector of Deployment
information and communication
GMF Taxonomy Classifications
Taxonomy DetailsKnown AI Goal
Image Cropping
Known AI Technology
Neural Network
Known AI Technical Failure
Generalization Failure
Potential AI Technical Failure
Distributional Bias, Incomplete Data Attribute Capture
CSETv1_Annotator-3 Taxonomy Classifications
Taxonomy DetailsHarm Distribution Basis
race, sex
Sector of Deployment
information and communication
Incident Reports
Reports Timeline
- View the original report at its source
- View the report at the Internet Archive
Twitter‘s algorithm for automatically cropping images attached to tweets often doesn’t focus on the important content in them. A bother, for sure, but it seems like a minor one on the surface. However, over the weekend, researchers found th…
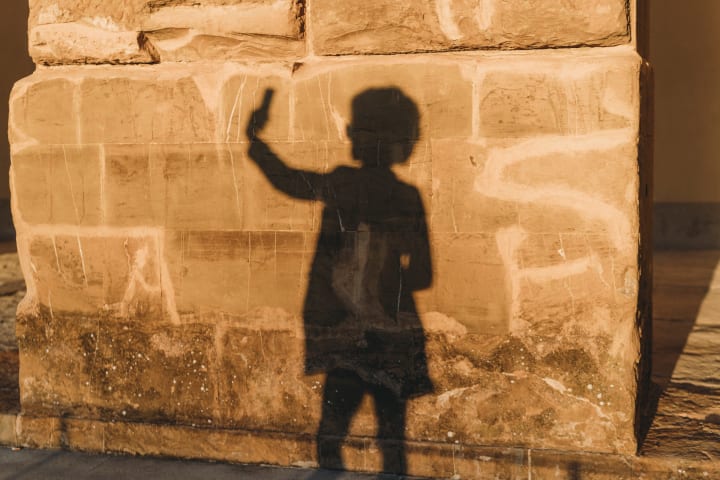
- View the original report at its source
- View the report at the Internet Archive
A study of 10,000 images found bias in what the system chooses to highlight. Twitter has stopped using it on mobile, and will consider ditching it on the web.
LAST FALL, CANADIAN student Colin Madland noticed that Twitter’s automatic croppi…
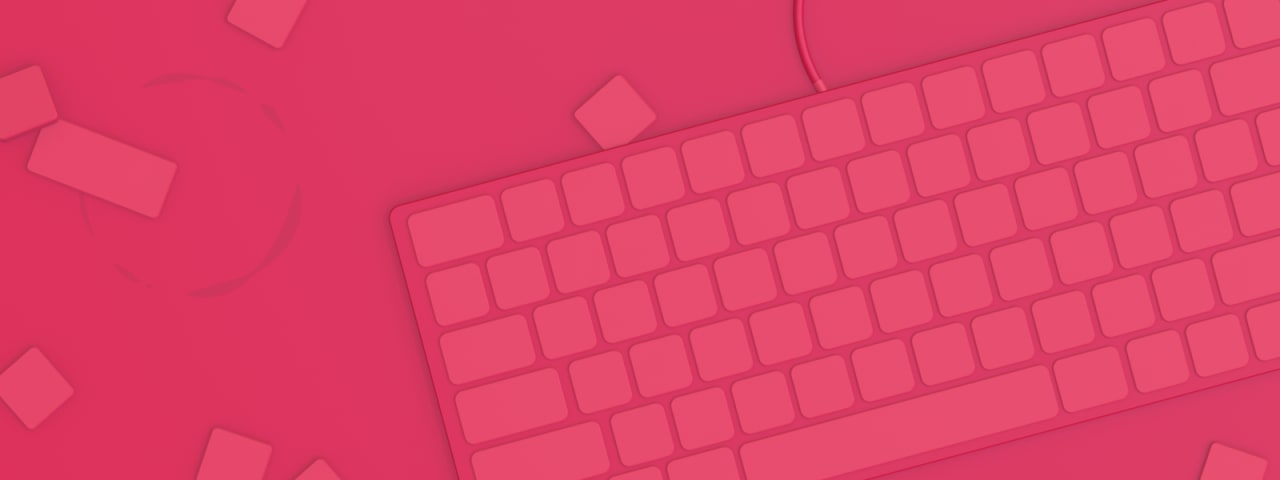
- View the original report at its source
- View the report at the Internet Archive
In October 2020, we heard feedback from people on Twitter that our image cropping algorithm didn’t serve all people equitably. As part of our commitment to address this issue, we also shared that we'd analyze our model again for bias. Over …
- View the original report at its source
- View the report at the Internet Archive
Twitter has laid out plans for a bug bounty competition with a difference. This time around, instead of paying researchers who uncover security issues, Twitter will reward those who find as-yet undiscovered examples of bias in its image-cro…
- View the original report at its source
- View the report at the Internet Archive
Twitter's first bounty program for AI bias has wrapped up, and there are already some glaring issues the company wants to address. CNET reports that grad student Bogdan Kulynych has discovered that photo beauty filters skew the Twitter sali…
Variants
Similar Incidents
Did our AI mess up? Flag the unrelated incidents
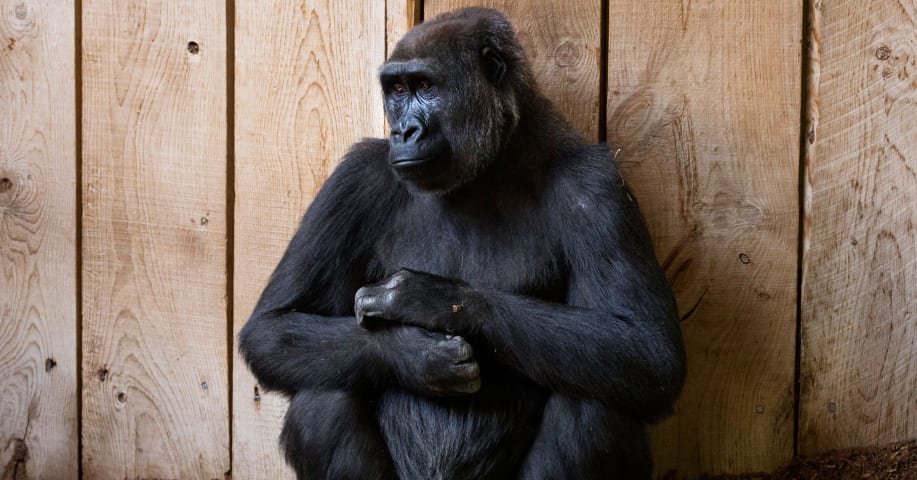
Images of Black People Labeled as Gorillas
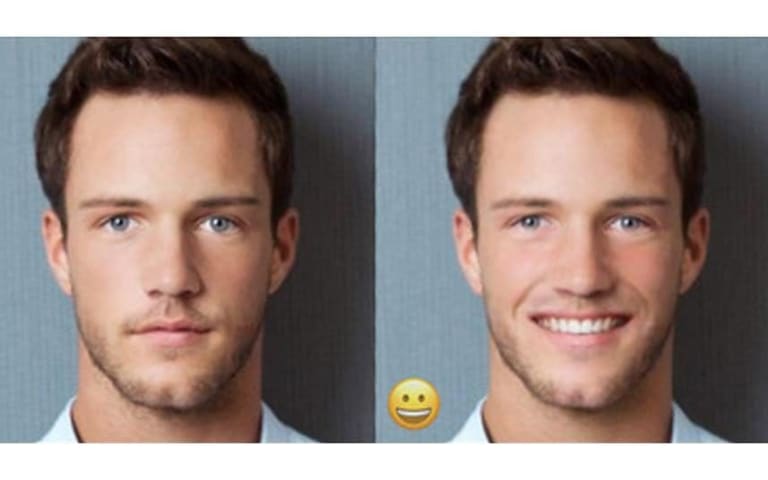
FaceApp Racial Filters
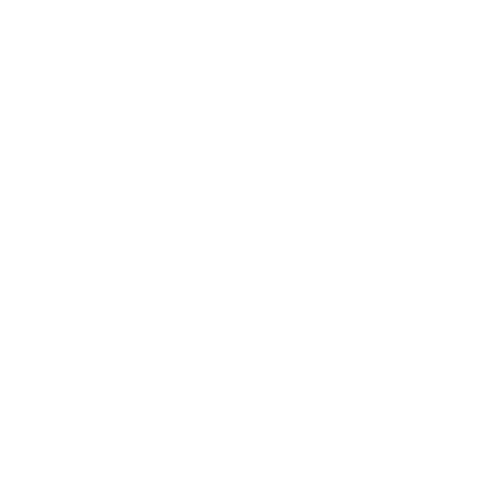
TayBot
Similar Incidents
Did our AI mess up? Flag the unrelated incidents
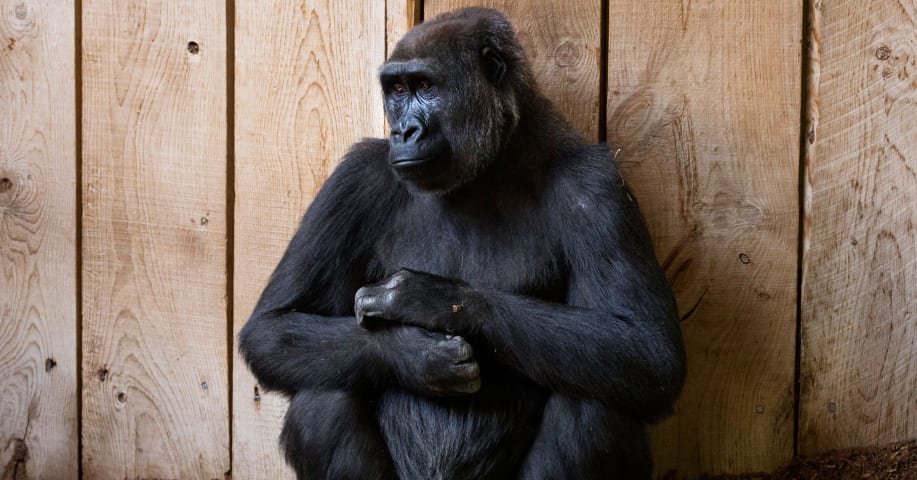
Images of Black People Labeled as Gorillas
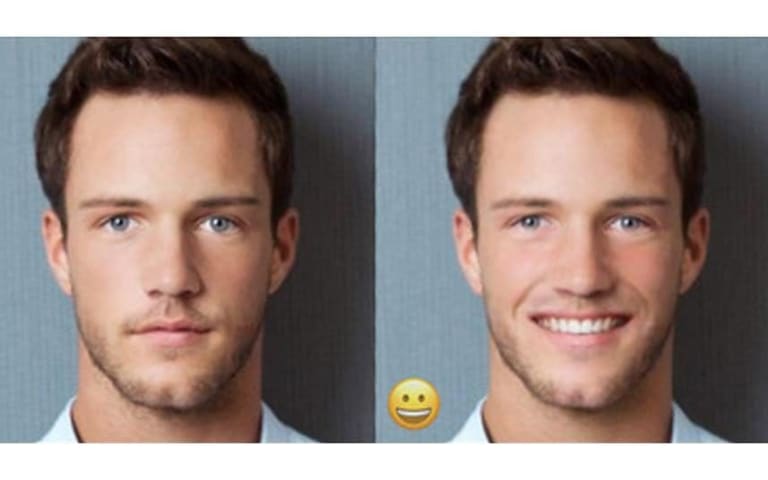
FaceApp Racial Filters
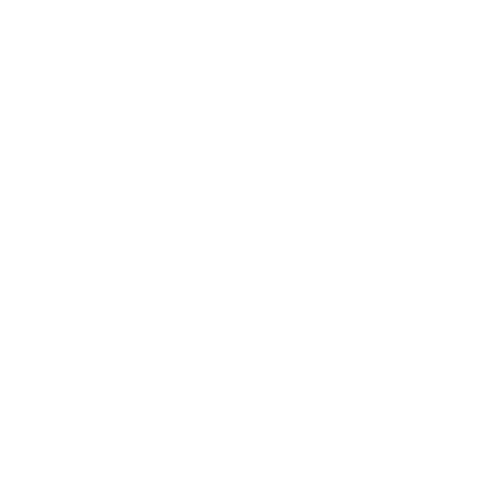