Entities
View all entitiesCSETv1 Taxonomy Classifications
Taxonomy DetailsIncident Number
29
Estimated Date
No
Lives Lost
0
Injuries
0
Estimated Harm Quantities
No
There is a potentially identifiable specific entity that experienced the harm
No
Risk Subdomain
7.3. Lack of capability or robustness
Risk Domain
- AI system safety, failures, and limitations
Entity
AI
Timing
Pre-deployment
Intent
Unintentional
Incident Reports
Reports Timeline
Drawing on Google/Google Books/Google Scholar/Libgen/LessWrong/Hacker News/Twitter, I have compiled a large number of variants of the story from various sources; below, in reverse chronological order by decade.
A similar thing happened here…
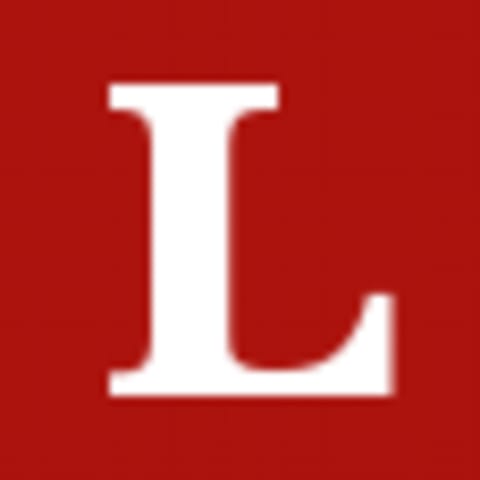
His team was working on running simulations of long-distance manned spaceflight. In particular, the goal of their simulations was to determine an algorithm that would optimally allocate food, water, and electricity to 3 crew members. The de…
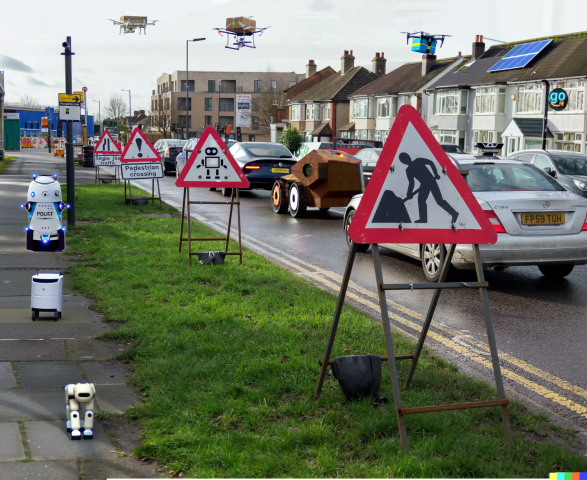
The following former incidents have been converted to "issues" following an update to the incident definition and ingestion criteria.
21: Tougher Turing Test Exposes Chatbots’ Stupidity
Description: The 2016 Winograd Schema Challenge highli…
Variants
Similar Incidents
Did our AI mess up? Flag the unrelated incidents
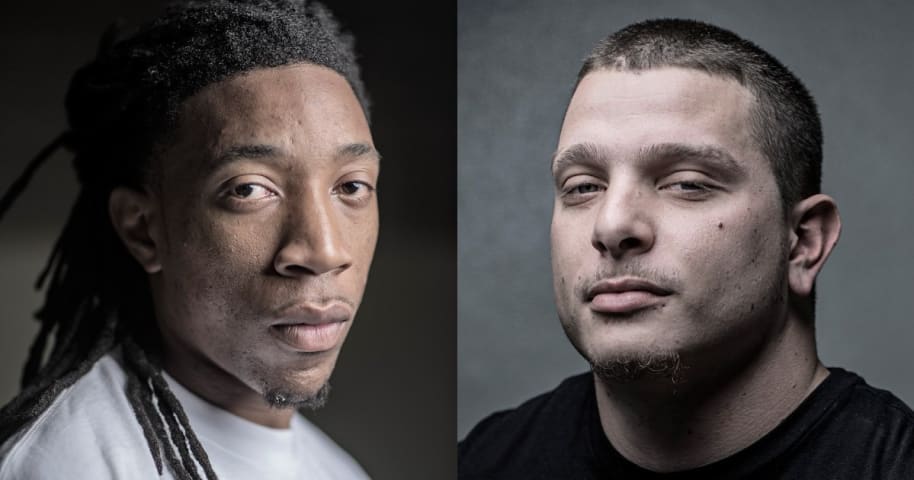
Northpointe Risk Models
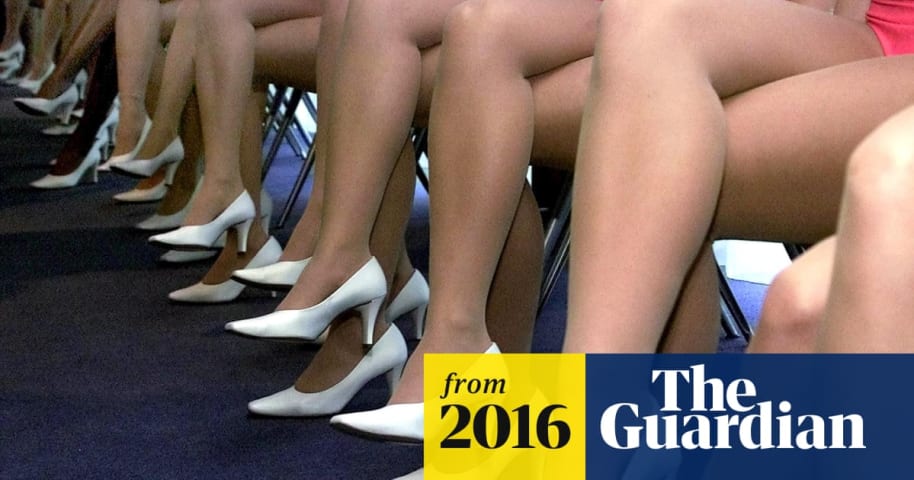
AI Beauty Judge Did Not Like Dark Skin
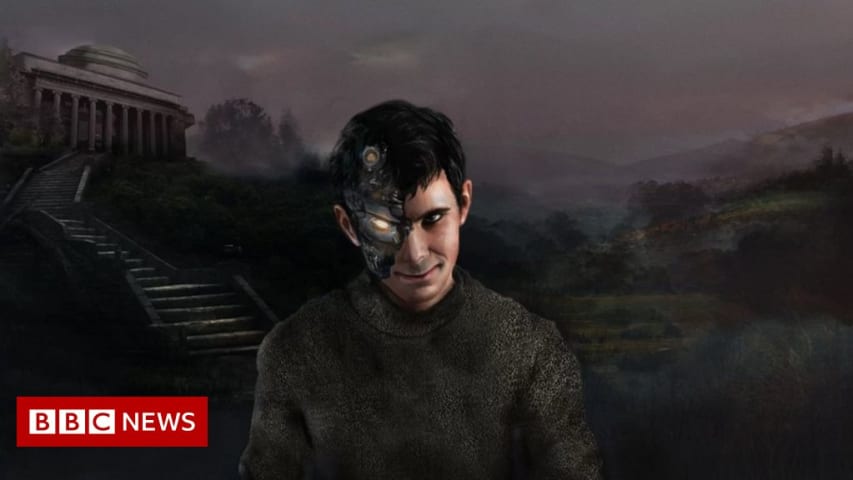
All Image Captions Produced are Violent
Similar Incidents
Did our AI mess up? Flag the unrelated incidents
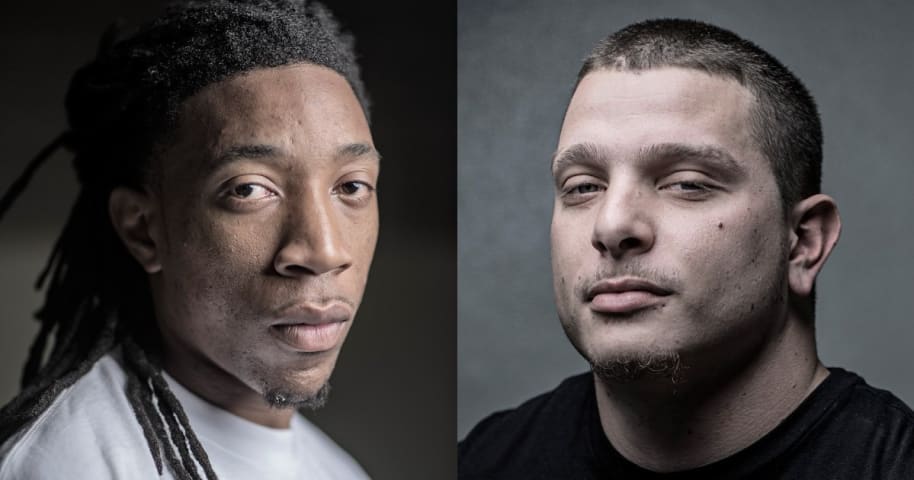
Northpointe Risk Models
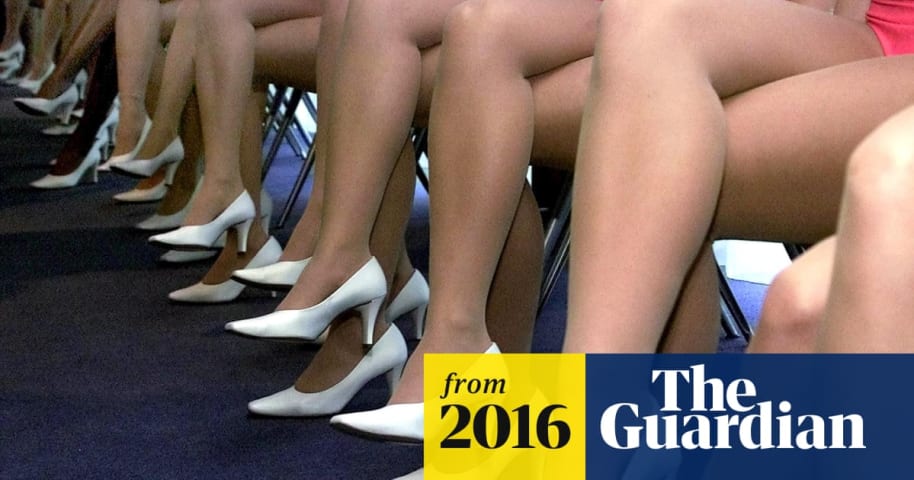
AI Beauty Judge Did Not Like Dark Skin
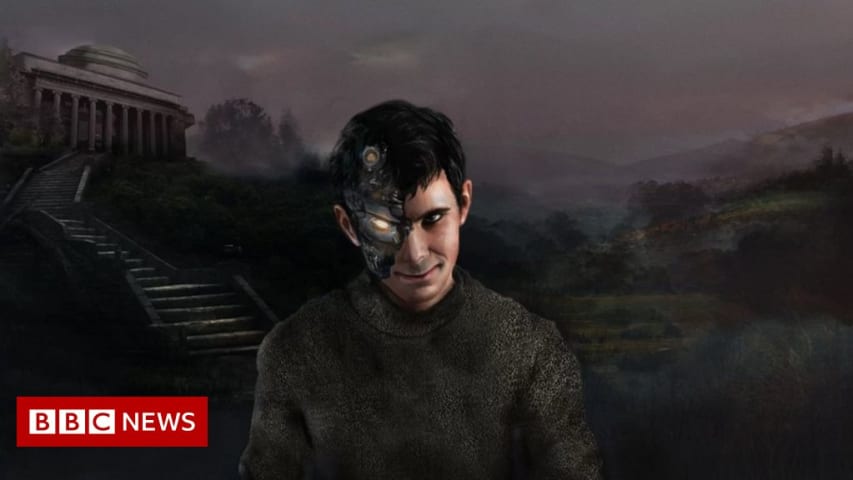