Entities
View all entitiesCSETv0 Taxonomy Classifications
Taxonomy DetailsPhysical System
Software only
Level of Autonomy
High
Nature of End User
Expert
Public Sector Deployment
No
Lives Lost
No
Intent
Unclear
GMF Taxonomy Classifications
Taxonomy DetailsKnown AI Goal Snippets
(Snippet Text: The Winograd Schema Challenge asks computers to make sense of sentences that are ambiguous but usually simple for humans to parse., Related Classifications: Question Answering)
CSETv1 Taxonomy Classifications
Taxonomy DetailsIncident Number
21
Estimated Date
No
Lives Lost
0
Injuries
0
Estimated Harm Quantities
No
There is a potentially identifiable specific entity that experienced the harm
No
Risk Subdomain
7.3. Lack of capability or robustness
Risk Domain
- AI system safety, failures, and limitations
Entity
AI
Timing
Pre-deployment
Intent
Unintentional
Incident Reports
Reports Timeline
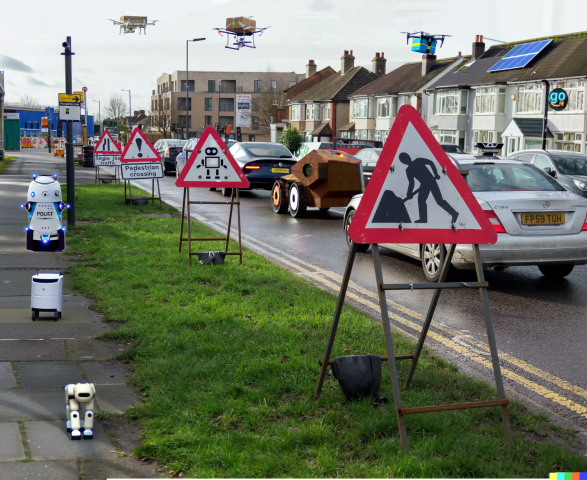
The following former incidents have been converted to "issues" following an update to the incident definition and ingestion criteria.
21: Tougher Turing Test Exposes Chatbots’ Stupidity
Description: The 2016 Winograd Schema Challenge highli…
Variants
Similar Incidents
Did our AI mess up? Flag the unrelated incidents
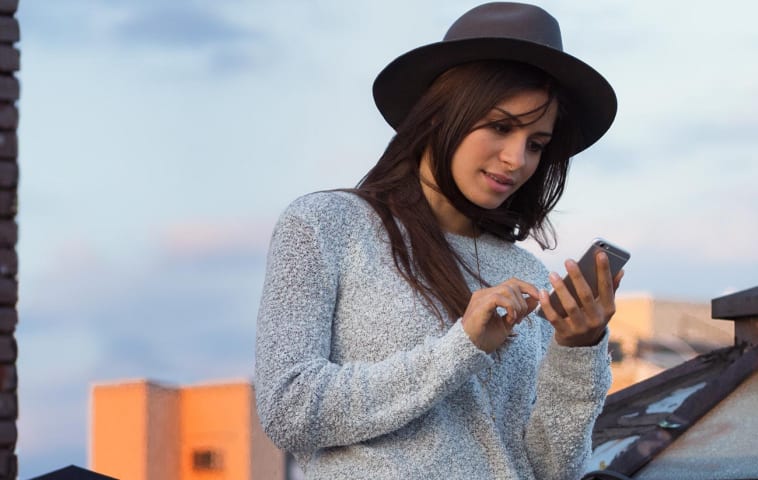
Inappropriate Gmail Smart Reply Suggestions
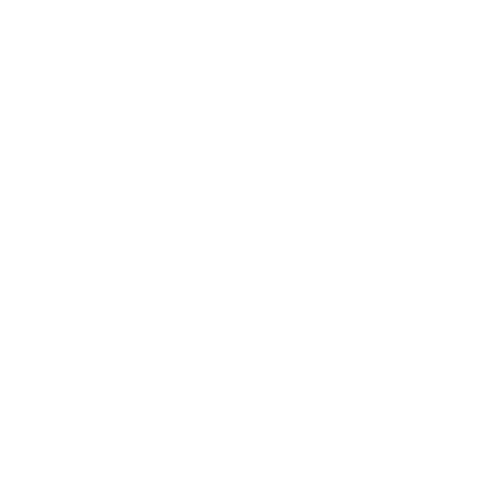
TayBot
Gender Biases in Google Translate
Similar Incidents
Did our AI mess up? Flag the unrelated incidents
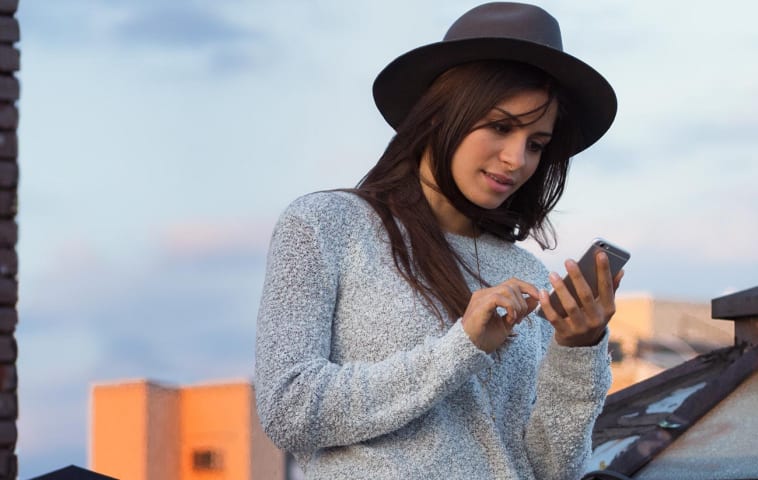
Inappropriate Gmail Smart Reply Suggestions
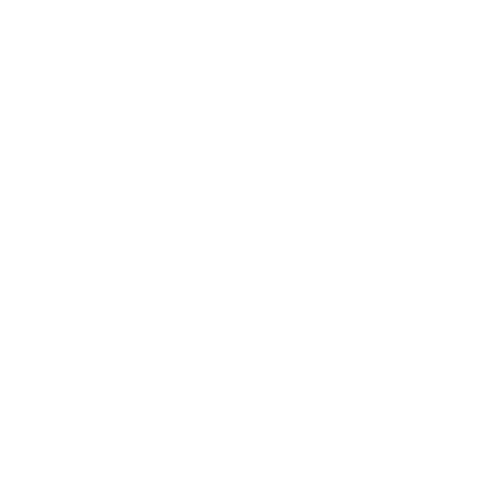