Entities
View all entitiesIncident Stats
Incident Reports
Reports Timeline
- View the original report at its source
- View the report at the Internet Archive
Allan Candelore had a problem with Tinder Plus prices, and made it known. He sued the company behind the dating app for discrimination. It was 2015.
In a California trial court, Tinder argued there was no problem. The company was charging u…
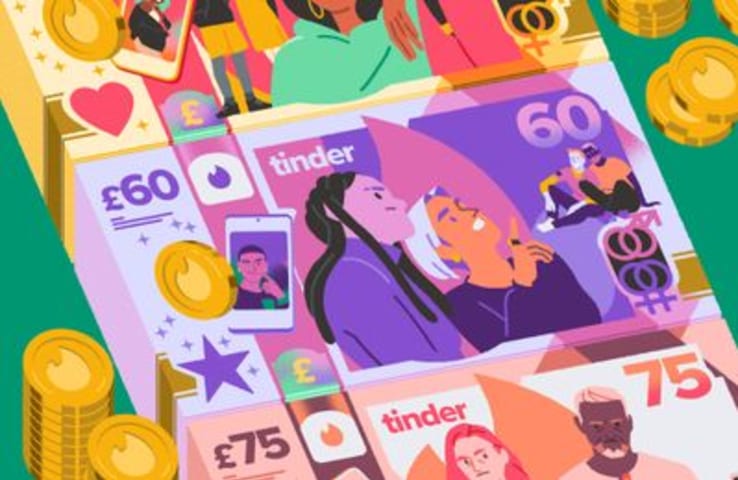
- View the original report at its source
- View the report at the Internet Archive
Over-30s pay more for Tinder Plus, Which? finds
A Which? investigation has revealed that market-leading dating app Tinder routinely charges over-30s more for its 'Plus' subscription.
UPDATE: Our original analysis also found that Tinder appe…
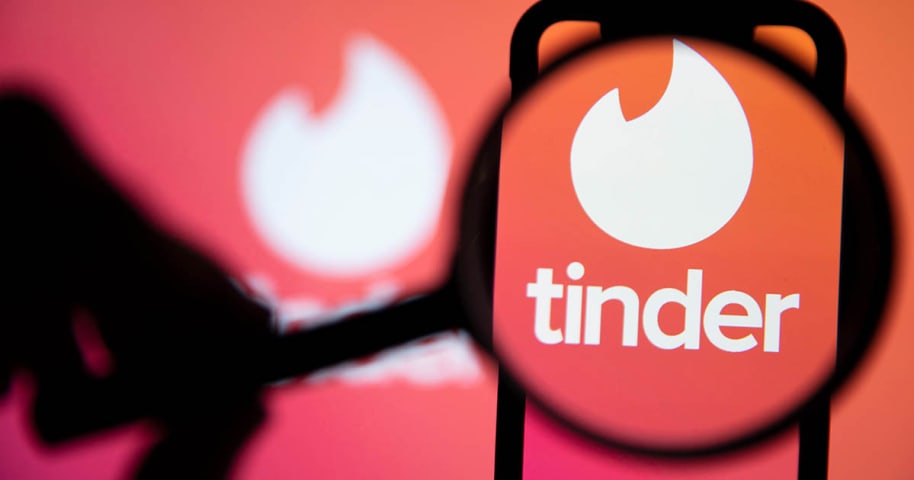
- View the original report at its source
- View the report at the Internet Archive
New research from Consumers International and the Mozilla Foundation has found that Tinder Plus users over thirty are paying 65 percent more than their younger counterparts on average to swipe right. The research looked at pricing from New …
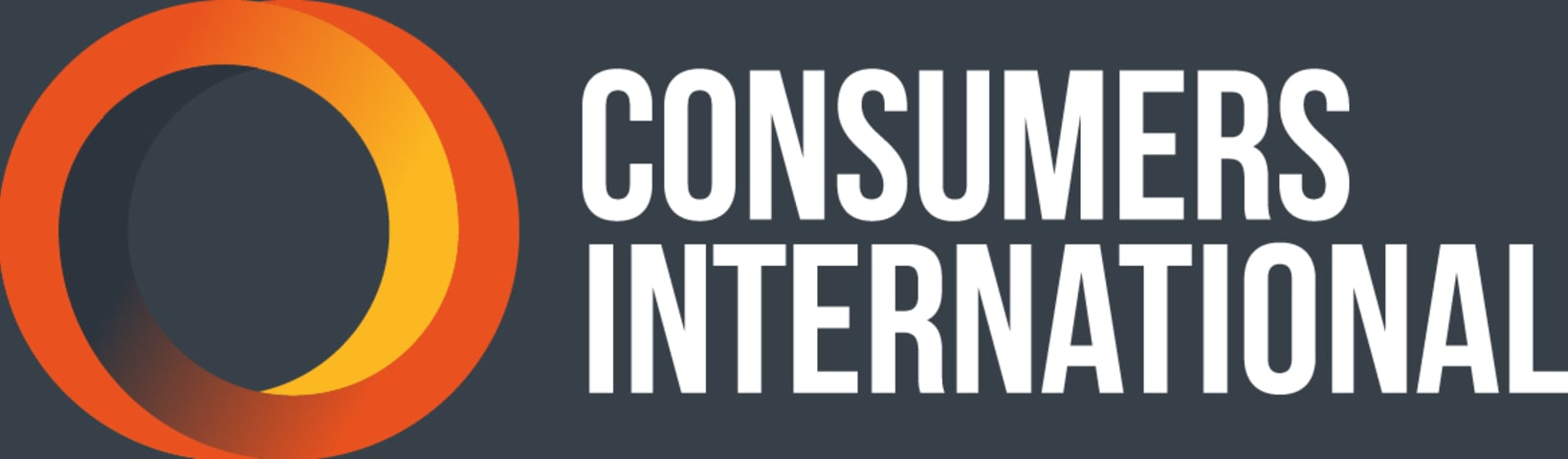
- View the original report at its source
- View the report at the Internet Archive
Background to Case Study
The primary objective of consumer organisations is the protection and promotion of consumer rights. Where possible, consumer organisations seek to combine efforts across countries to partner, leverage, and learn tog…