Entités
Voir toutes les entitésClassifications de taxonomie CSETv0
Détails de la taxonomiePhysical System
Software only
Level of Autonomy
High
Nature of End User
Expert
Public Sector Deployment
No
Lives Lost
No
Intent
Unclear
Classifications de taxonomie GMF
Détails de la taxonomieKnown AI Goal Snippets
(Snippet Text: The Winograd Schema Challenge asks computers to make sense of sentences that are ambiguous but usually simple for humans to parse., Related Classifications: Question Answering)
Classifications de taxonomie CSETv1
Détails de la taxonomieIncident Number
21
Estimated Date
No
Lives Lost
0
Injuries
0
Estimated Harm Quantities
No
There is a potentially identifiable specific entity that experienced the harm
No
Risk Subdomain
7.3. Lack of capability or robustness
Risk Domain
- AI system safety, failures, and limitations
Entity
AI
Timing
Pre-deployment
Intent
Unintentional
Rapports d'incidents
Chronologie du rapport
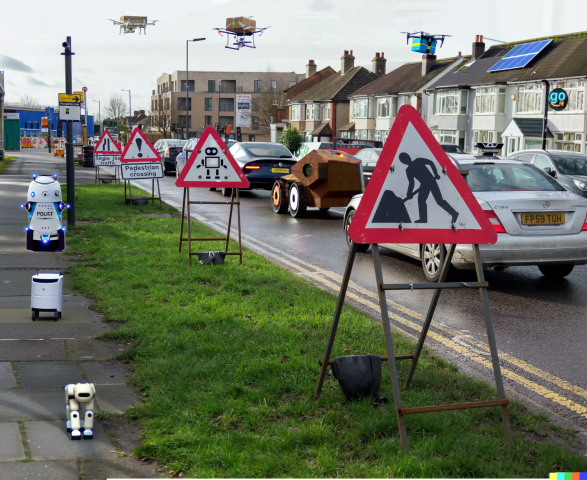
Les anciens incidents suivants ont été convertis en "issues" suite à une mise à jour de la définition d'incident et critères d'ingestion.
21 : Un test de Turing plus difficile révèle la stupidité des chatbots
Description : Le Winograd Schem…
Variantes
Incidents similaires
Did our AI mess up? Flag the unrelated incidents
Incidents similaires
Did our AI mess up? Flag the unrelated incidents