Incident 84: De minuscules changements permettent aux fausses déclarations sur la COVID-19 et le vote d'échapper aux vérifications des faits sur Facebook
Entités
Voir toutes les entitésClassifications de taxonomie CSETv1
Détails de la taxonomieIncident Number
84
AI Tangible Harm Level Notes
3.3 - Although there was no tangible harm, the AI was linked to the adverse outcome described in the incident.
Notes (special interest intangible harm)
AI failed to prevent the spread of misinformation
Special Interest Intangible Harm
yes
Date of Incident Year
2019
Date of Incident Month
10
Classifications de taxonomie CSETv0
Détails de la taxonomieProblem Nature
Robustness
Physical System
Software only
Level of Autonomy
Medium
Nature of End User
Amateur
Public Sector Deployment
No
Data Inputs
User posts
Risk Subdomain
7.3. Lack of capability or robustness
Risk Domain
- AI system safety, failures, and limitations
Entity
AI
Timing
Post-deployment
Intent
Unintentional
Rapports d'incidents
Chronologie du rapport
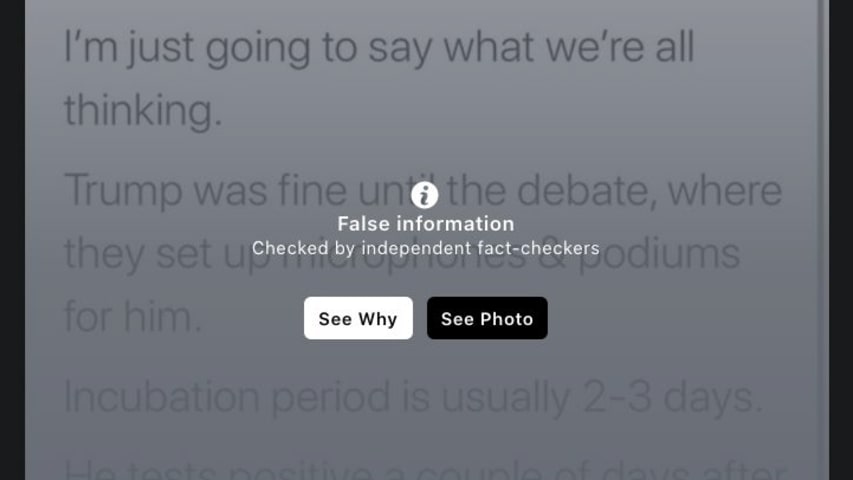
Quelque chose d'aussi simple que de changer la police d'un message ou de recadrer une image peut suffire à contourner les défenses de Facebook contre les canulars et les mensonges.
Une nouvelle analyse du groupe de défense international Ava…
Variantes
Incidents similaires
Did our AI mess up? Flag the unrelated incidents
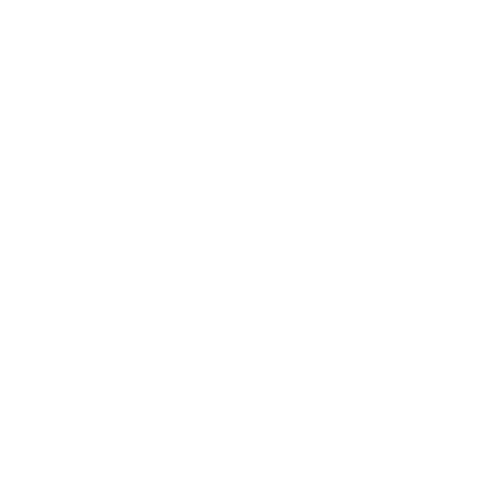
Wikipedia Vandalism Prevention Bot Loop
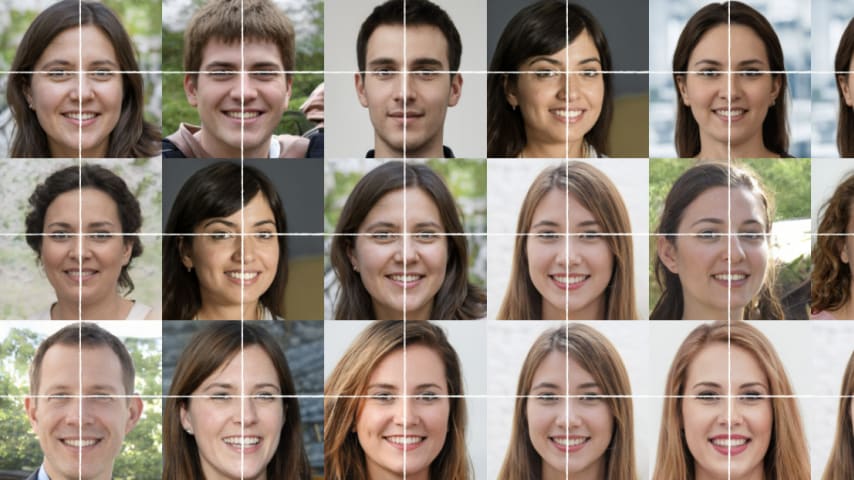
Fake LinkedIn Profiles Created Using GAN Photos
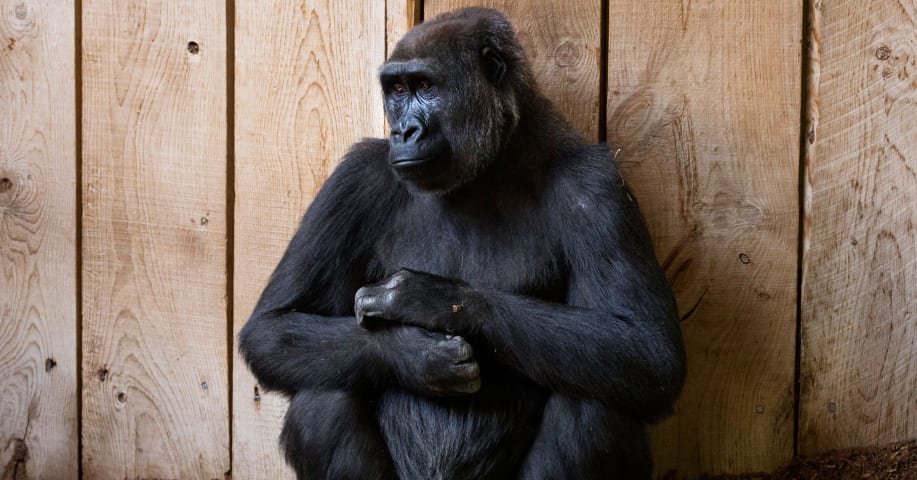
Images of Black People Labeled as Gorillas
Incidents similaires
Did our AI mess up? Flag the unrelated incidents
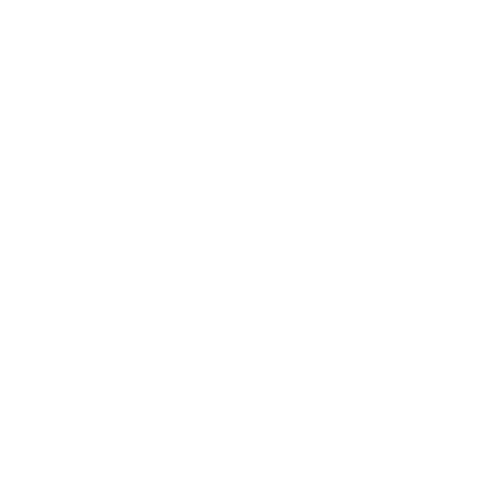
Wikipedia Vandalism Prevention Bot Loop
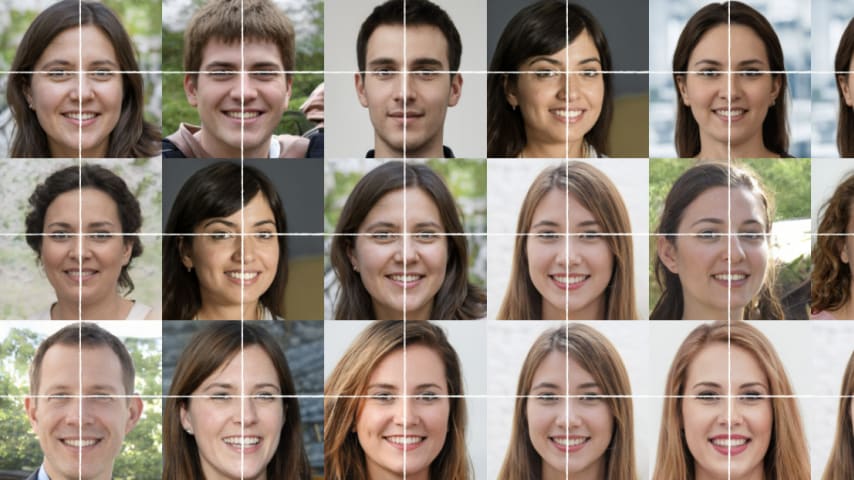
Fake LinkedIn Profiles Created Using GAN Photos
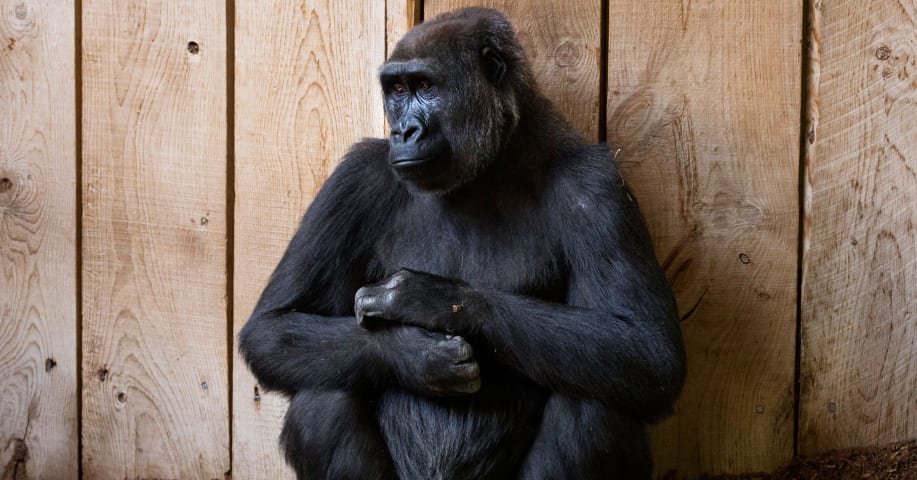