CSETv1 分類法のクラス
分類法の詳細Incident Number
10
Special Interest Intangible Harm
no
Date of Incident Year
2014
Estimated Date
Yes
Multiple AI Interaction
no
Embedded
no
CSETv0 分類法のクラス
分類法の詳細Public Sector Deployment
No
Infrastructure Sectors
Food and agriculture
Lives Lost
No
Intent
Unclear
Near Miss
Unclear/unknown
Ending Date
2015-01-01
GMF 分類法のクラス
分類法の詳細Potential AI Technology Snippets
(Snippet Text: “You’re waiting on your job to control your life,” she said, with the scheduling software used by her employer dictating everything from “how much sleep Gavin will get to what groceries I’ll be able to buy this month.”, Related Classifications: Regression), (Snippet Text: In a follow-up piece, the author, Jodi Kantor, points directly to Kronos' scheduling software as the root of the problem., Related Classifications: Diverse Data)
Risk Subdomain
5.2. Loss of human agency and autonomy
Risk Domain
- Human-Computer Interaction
Entity
AI
Timing
Post-deployment
Intent
Unintentional
インシデントレポート
レポートタイムライン
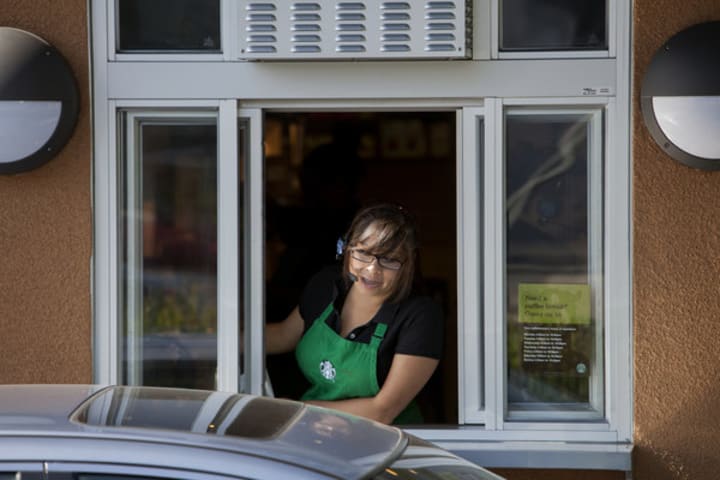
SAN DIEGO — In a typical last-minute scramble, Jannette Navarro, a 22-year-old Starbucks barista and single mother, scraped together a plan for surviving the month of July without setting off family or financial disaster.
In contrast to the…
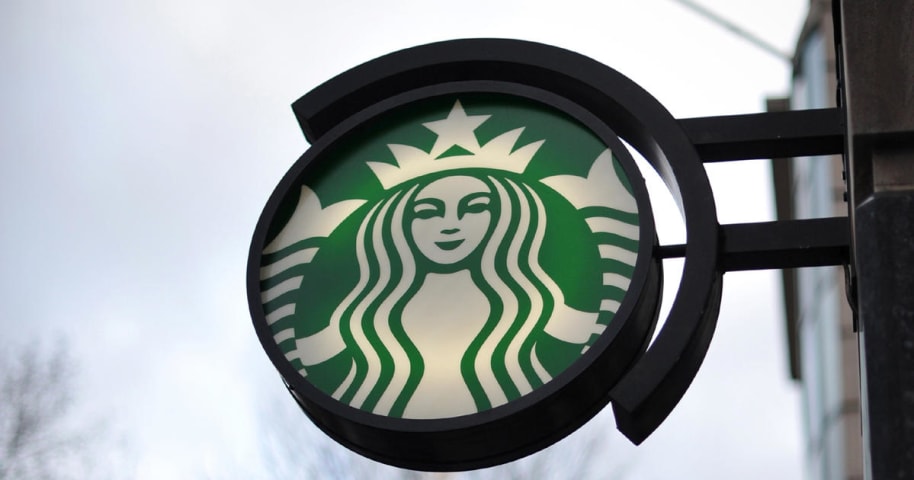
Liberte Locke, a 32-year-old "barista" at a Starbucks (SBUX) in New York City, is fed up.
"Starbucks' attitude is that there's always someone else who can do the job," she said in running through her complaints about life at the java giant.…
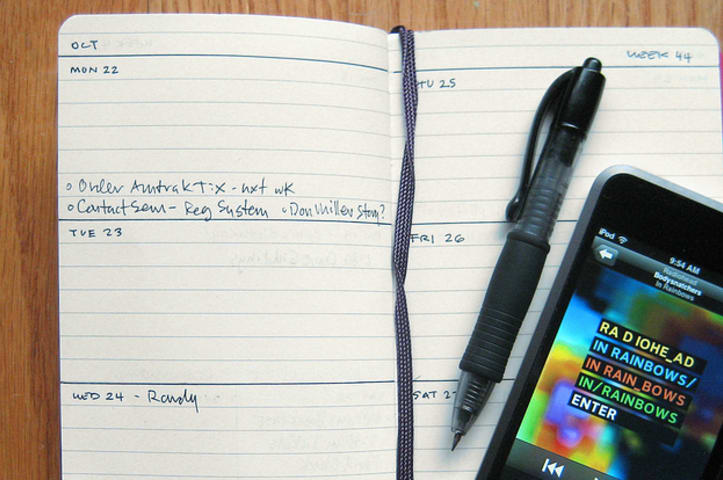
In April, the New York attorney general's office launched an investigation into the scheduling practices of 13 national retail chains, distributing a letter to the Gap, Target, J.C. Penney, and 10 other companies. The letter asked, among ot…
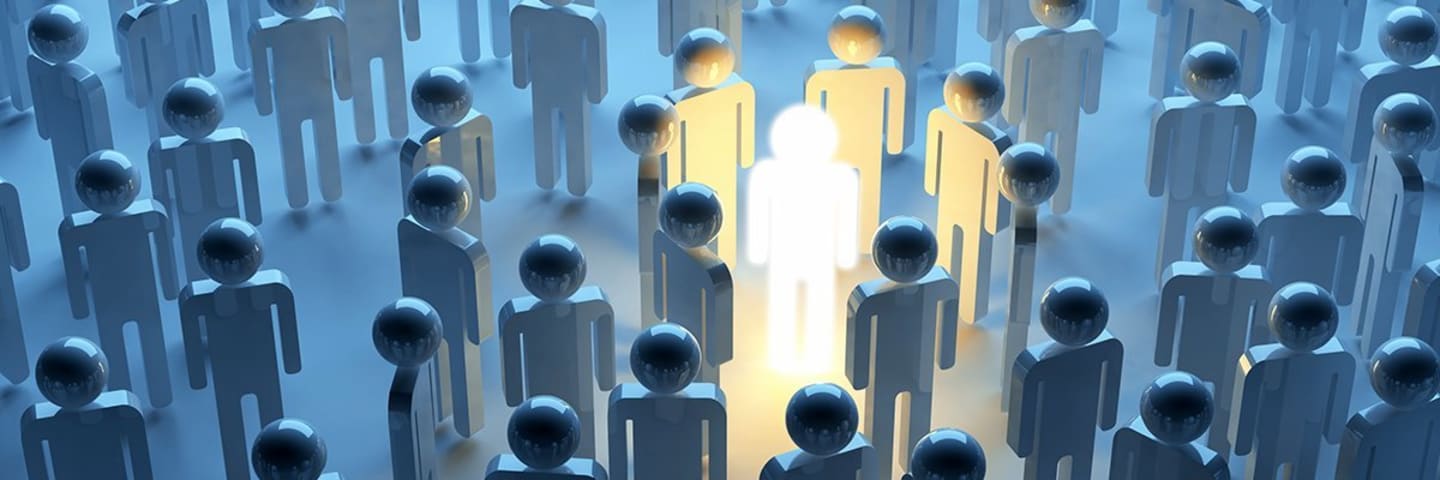
Caitlin O'Reilly-Green, a barista at an Atlanta Starbucks, says a manager blames her erratic hours on the staff scheduling software used by the giant coffee chain.
"When you try to bring up issues with it, they just kind of blame it on the …
A 2015 nationwide survey of Starbucks workers reveals that the company is not living up to its commitment to provide predictable, sustainable schedules to its workforce. Starbucks’ frontline employees bear the brunt of the management impera…
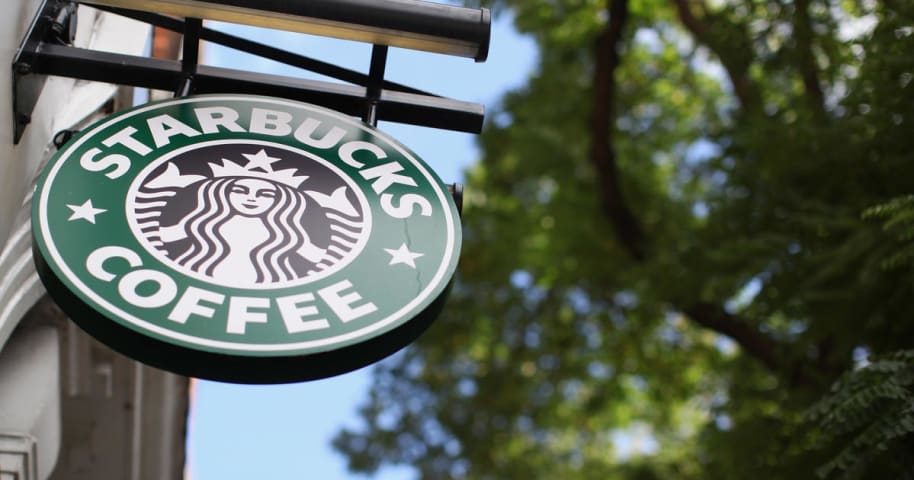
For Starbucks (SBUX) barista Kylei Weisse, working at the coffee chain helps him secure health insurance and some extra money while he studies at Georgia Perimeter College. What it doesn't provide is the kind of stable schedule that the com…
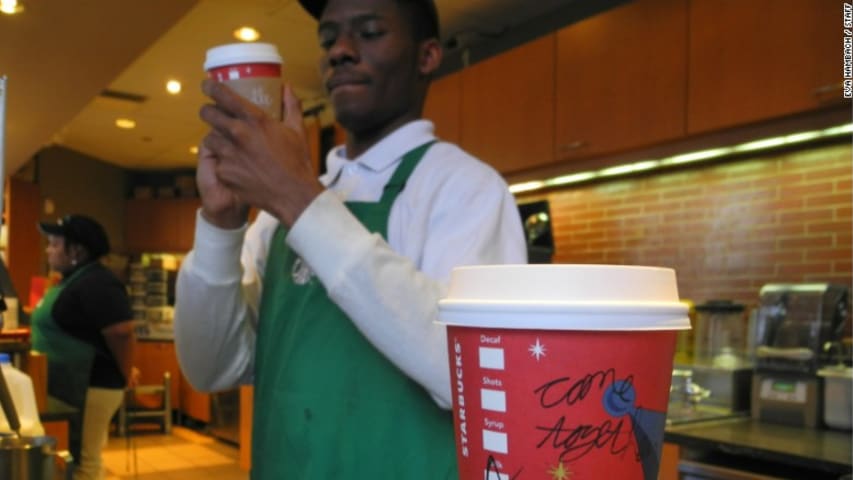
An internal memo from a Starbucks executive this week urged store managers to "go the extra mile" to improve workers' schedules.
The letter was distributed on Tuesday and refers to a New York Times story that was set to be published the fol…
On Tuesday, I wrote about Starbucks baristas and other food service workers who marched downtown at sunrise to call for fair scheduling from their employers. Workers said they often get little notice of when they're scheduled to work and th…
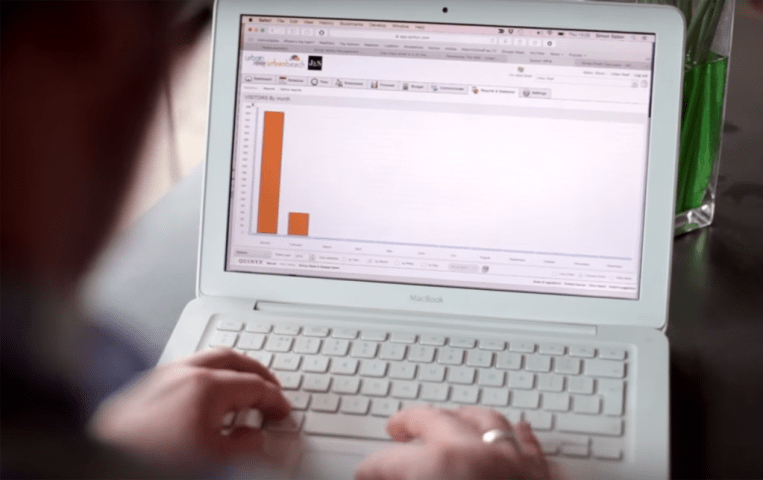
The best schedules deliver two things; they ensure a business maximises profit while at the same time keeping the workforce happy and motivated.
At first glance, these two outcomes seem to be coming from opposite sides of the spectrum. To m…
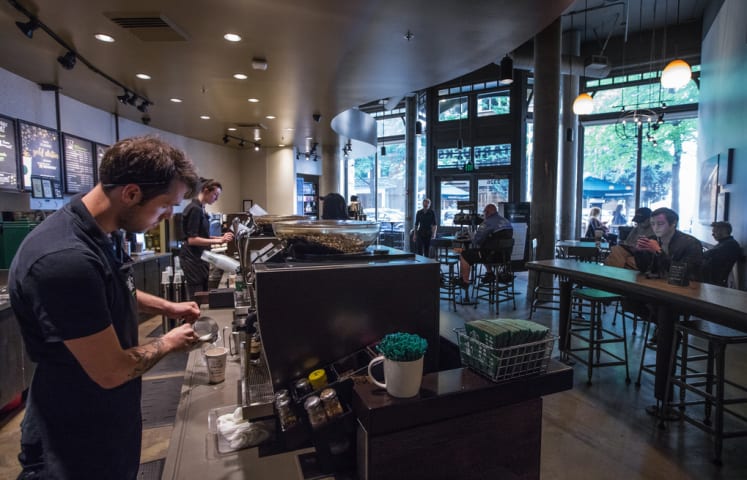
Originally published on Seattle Times on June 4, 2016 at 8:00 am
The company took a lot of heat in 2014 when The New York Times described scheduling practices that made some employees miserable. But the coffee giant says its policies and so…
バリアント
よく似たインシデント
Did our AI mess up? Flag the unrelated incidents
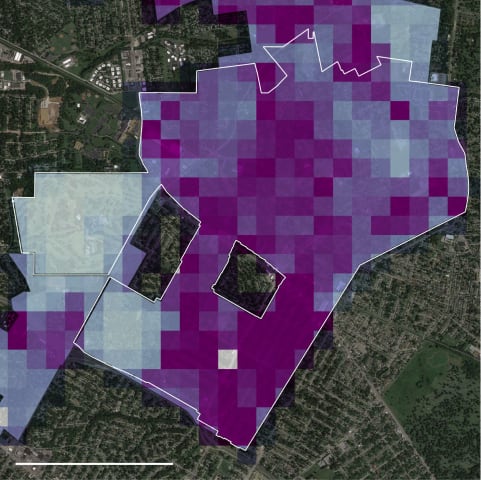
Policing the Future
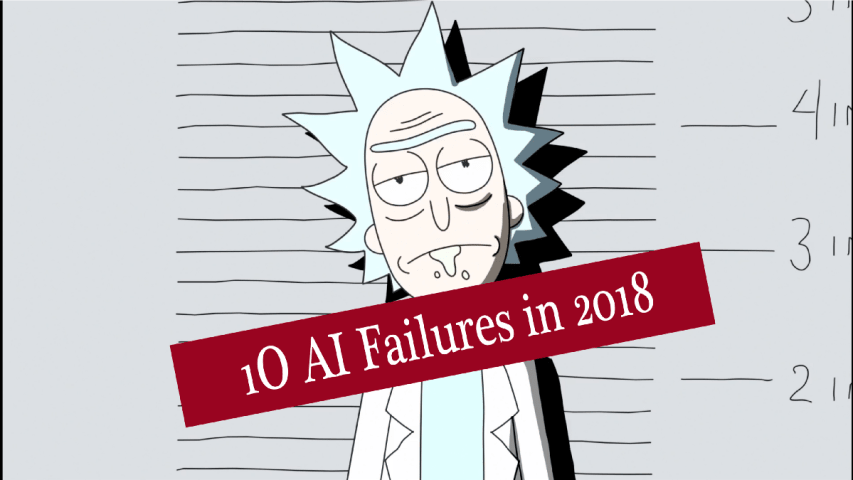
2018 in Review: 10 AI Failures
よく似たインシデント
Did our AI mess up? Flag the unrelated incidents
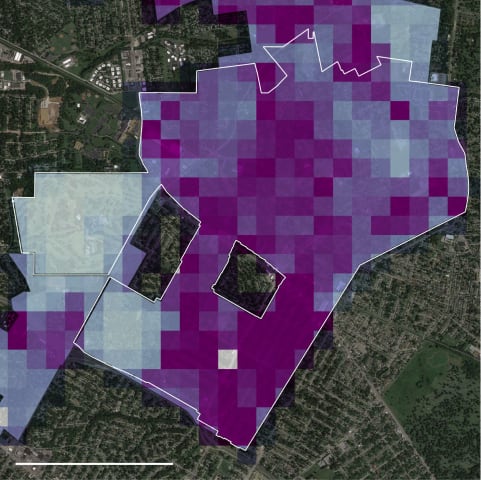
Policing the Future
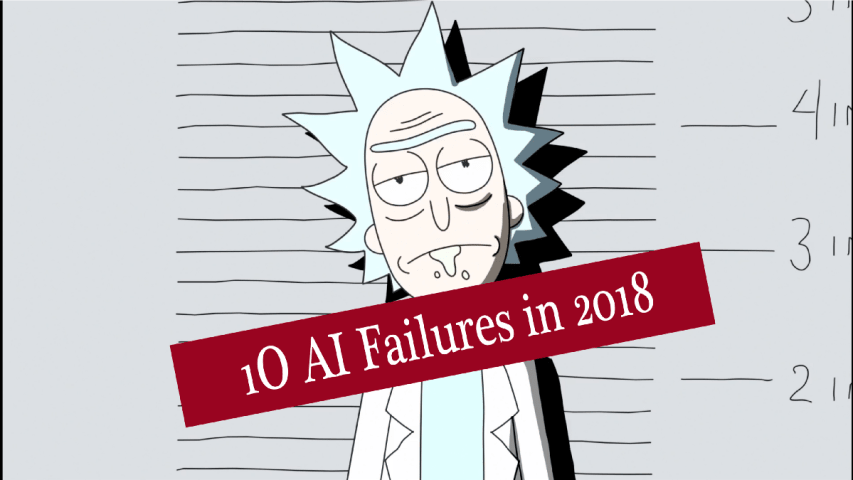