Registro de citas para el Incidente 21
Entidades
Ver todas las entidadesEstadísticas de incidentes
Clasificaciones de la Taxonomía CSETv0
Detalles de la TaxonomíaFull Description
The Winograd Schema Challenge in 2016 highlighted shortcomings of an artificially intelligent system's ability to understand context. The Challenge is designed to present ambiguous sentences and ask AI systems to decipher them. In the Winograd Scheme Challenge, the two winning entries were successful 48% of the time, while random chance was correct 45% of the time. Quan Liu of the University of Science and Technology of China (partnering with University of Toronto and National Research Council of Canada) and Nicos Isaak of the Open University of Cyprus presented the most successful systems. It is notable that Google and Facebook did not participate.
Short Description
The 2016 Winograd Schema Challenge highlighted how even the most successful AI systems entered into the Challenge were only successful 3% more often than random chance.
Severity
Unclear/unknown
AI System Description
Artificially intelligent systems meant to understand ambiguous English sentences.
Sector of Deployment
Professional, scientific and technical activities
Relevant AI functions
Perception, Cognition, Action
Location
New York, NY
Named Entities
Winograd Schema Challenge, University of Science and Technology of China, Quan Liu, University of Toronto, National Research Council of Canada, Nicos Isaak, Open University of Cyprus
Technology Purveyor
Quan Liu, Nicos Isaak
Beginning Date
2016-01-01T00:00:00.000Z
Ending Date
2016-01-01T00:00:00.000Z
Near Miss
Unclear/unknown
Intent
Unclear
Lives Lost
No
Clasificaciones de la Taxonomía GMF
Detalles de la TaxonomíaKnown AI Goal
Question Answering
Known AI Technology
Language Modeling, Distributional Learning
Potential AI Technology
Transformer
Potential AI Technical Failure
Generalization Failure, Dataset Imbalance, Underfitting, Context Misidentification
Clasificaciones de la Taxonomía CSETv1
Detalles de la TaxonomíaInformes del Incidente
Cronología de Informes
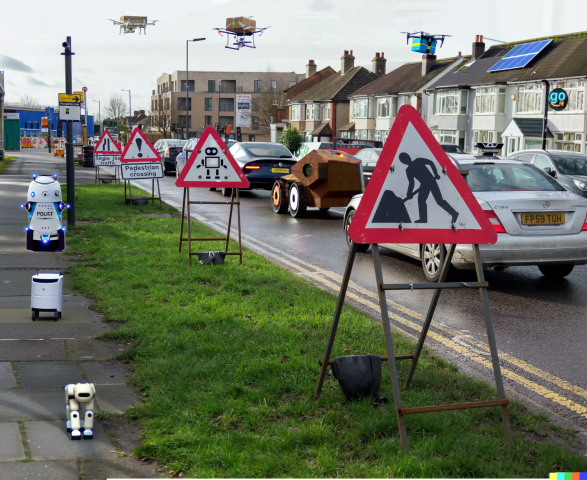
- Ver el informe original en su fuente
- Ver el informe en el Archivo de Internet
Los siguientes incidentes anteriores se han convertido a "problemas" luego de una actualización de definición de incidentes y criterios de ingestión.
21: Una prueba de Turing más dura expone la estupidez de los chatbots
Descripción: El Wino…
Variantes
Incidentes Similares
Did our AI mess up? Flag the unrelated incidents
Incidentes Similares
Did our AI mess up? Flag the unrelated incidents