Entidades
Ver todas las entidadesEstadísticas de incidentes
Clasificaciones de la Taxonomía CSETv1
Detalles de la TaxonomíaIncident Number
114
Special Interest Intangible Harm
yes
Notes (AI special interest intangible harm)
The ACLU's test demonstrated Rekognition's disproportionate inaccuracy on the faces of people of color.
Date of Incident Year
2018
Date of Incident Month
07
Estimated Date
No
Clasificaciones de la Taxonomía CSETv1_Annotator-1
Detalles de la TaxonomíaIncident Number
114
Special Interest Intangible Harm
yes
Date of Incident Year
2018
Date of Incident Month
07
Estimated Date
No
Multiple AI Interaction
no
Clasificaciones de la Taxonomía CSETv1_Annotator-3
Detalles de la TaxonomíaIncident Number
114
Special Interest Intangible Harm
no
Informes del Incidente
Cronología de Informes
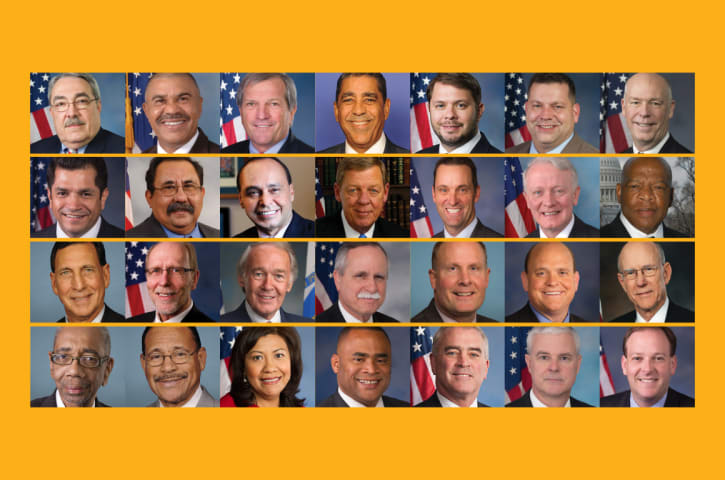
- Ver el informe original en su fuente
- Ver el informe en el Archivo de Internet
La tecnología de vigilancia facial de Amazon es objeto de una creciente oposición en todo el país y, en la actualidad, hay 28 motivos más de preocupación. En una prueba que la ACLU realizó recientemente de la herramienta de reconocimiento f…
Variantes
Incidentes Similares
Did our AI mess up? Flag the unrelated incidents
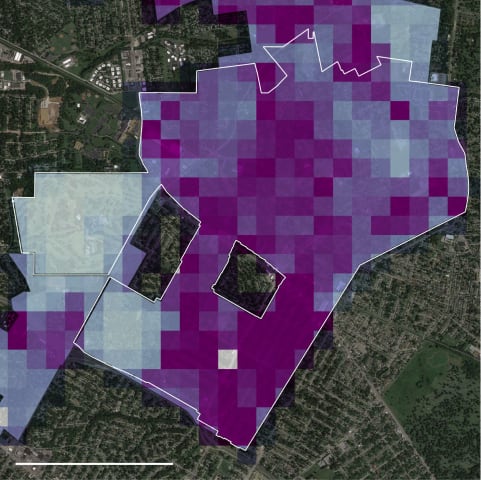
Policing the Future
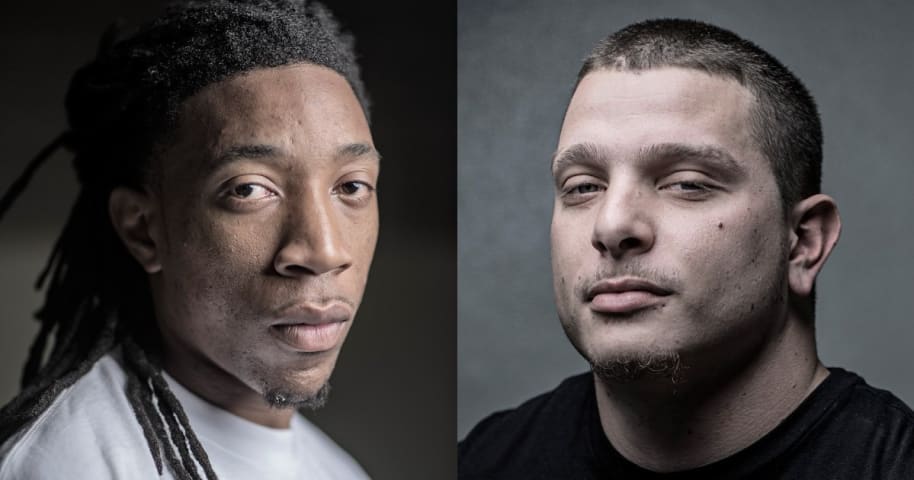
Machine Bias - ProPublica
Incidentes Similares
Did our AI mess up? Flag the unrelated incidents
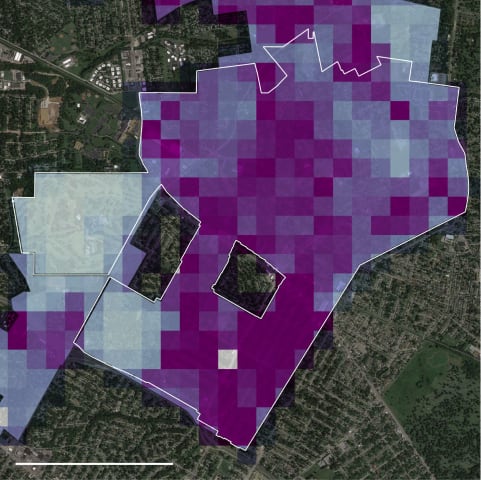
Policing the Future
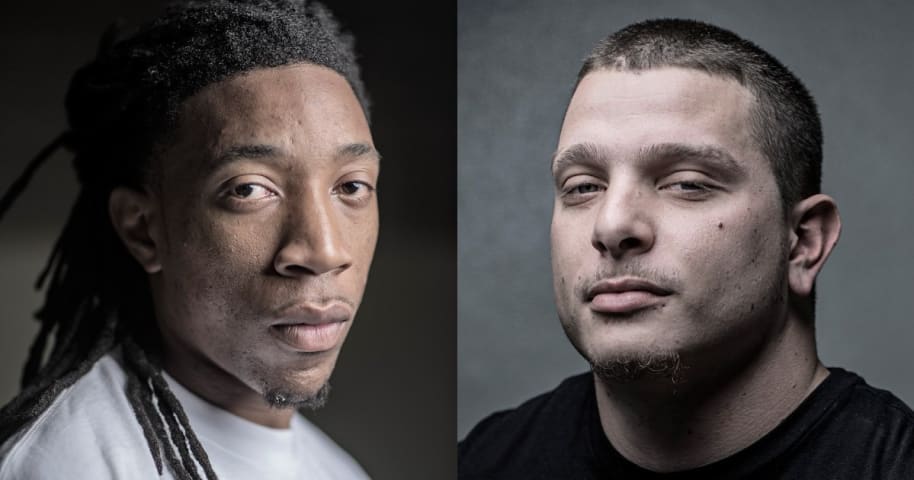