インシデントのステータス
CSETv0 分類法のクラス
分類法の詳細Full Description
In November 2019, customers of Goldman-Sachs and Apple's Apple Card, the first credit offering by Goldman-Sachs, claimed that there was gender discrimination in the credit assessment algorithm that distributes credit lines, with men receiving significantly higher credit limits than women with equal credit qualifications. Apple co-founder Steve Wozniak confirmed this also happened with him and his wife and the New York Department for Financial Services have launched an investigation regarding the discrimination claim. In response to this incident, Goldman Sachs made a statement that it has not and will never make decisions based on factors like gender, race, age, sexual orientation or any other legally prohibited factors when determining credit worthiness.
Short Description
In November 2019, Apple Card clients claimed that the credit assessment algorithm possesses a gender bias in favor of men.
Severity
Minor
Harm Distribution Basis
Sex
Harm Type
Financial harm
AI System Description
Goldman-Sachs uses a credit assessment algorithm that factors credit score, credit report, and reported income to determine credit lines for clients
System Developer
Goldman-Sachs
Sector of Deployment
Financial and insurance activities
Relevant AI functions
Perception, Cognition, Action
AI Techniques
machine learning, daya analytics
AI Applications
data analytics, recommendation engine, decision support
Location
United States
Named Entities
Goldman Sachs, Apple Card, Apple, Steve Wozniak, New York Department of Financial Services
Technology Purveyor
Apple, Goldman-Sachs
Beginning Date
11/2019
Ending Date
11/2019
Near Miss
Harm caused
Intent
Accident
Lives Lost
No
Laws Implicated
The Equal Credit Opportunity Act (ECOA) prohibits credit discrimination on the basis of race, color, religion, national origin, sex, marital status, age, or because you get public assistance.
Data Inputs
credit score, credit report, reported income
インシデントレポート
レポートタイムライン
- 情報源として元のレポートを表示
- インターネットアーカイブでレポートを表示
What started with a viral Twitter thread metastasized into a regulatory investigation of Goldman Sachs’ credit card practices after a prominent software developer called attention to differences in Apple Card credit lines for male and femal…
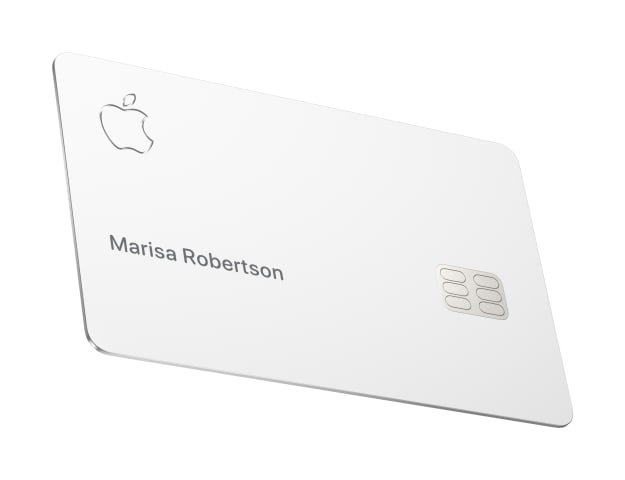
- 情報源として元のレポートを表示
- インターネットアーカイブでレポートを表示
The algorithm responsible for credit decisions for the Apple Card is giving females lower credit limits than equally qualified males. Those are the allegations that began spreading as consumers took to social media with complaints about App…
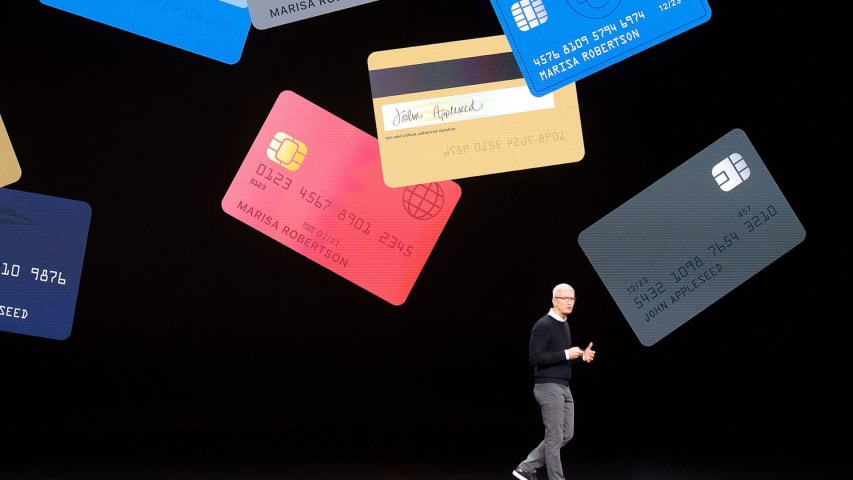
- 情報源として元のレポートを表示
- インターネットアーカイブでレポートを表示
When tech entrepreneur David Heinmeier Hansson recently took to Twitter saying the Apple Card gave him a credit limit that was 20 times higher than his wife's, despite the fact that she had a higher credit score, it may have been the first …
- 情報源として元のレポートを表示
- インターネットアーカイブでレポートを表示
US regulators are investigating whether Apple’s credit card, launched in August, is biased against women. Software engineer David Heinemeier Hansson reported on social media that Apple had offered him a spending limit 20 times higher than h…
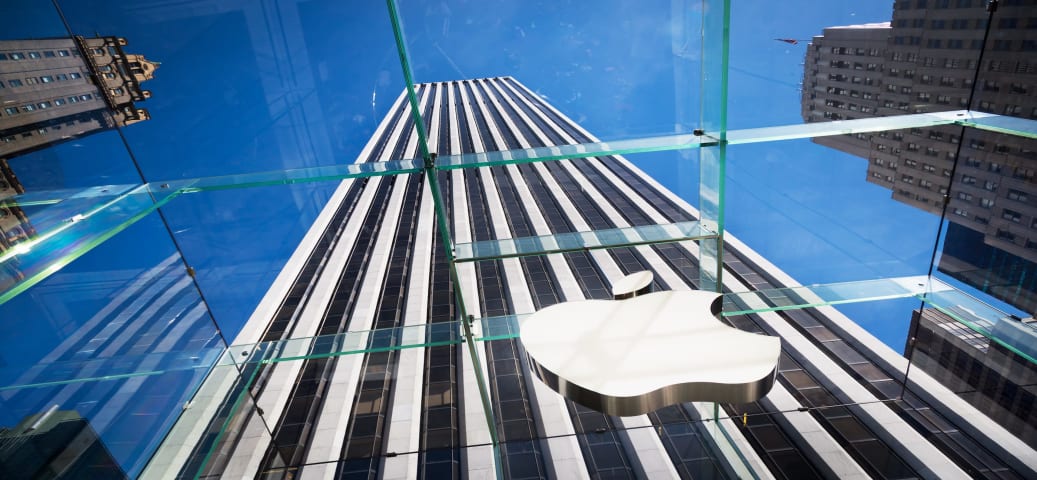
- 情報源として元のレポートを表示
- インターネットアーカイブでレポートを表示
The possibility that Apple Card applicants were subject to gender bias opens a new frontier for the financial services sector in which regulators are largely absent, argues Karen Mills.
In late August, the Apple Card debuted with a minimali…
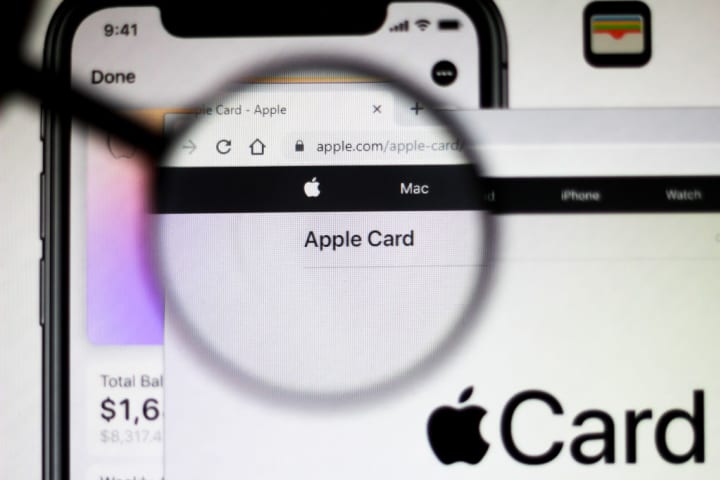
- 情報源として元のレポートを表示
- インターネットアーカイブでレポートを表示
Advocates of algorithmic justice have begun to see their proverbial “days in court” with legal investigations of enterprises like UHG and Apple Card. The Apple Card case is a strong example of how current anti-discrimination laws fall short…
バリアント
よく似たインシデント
Did our AI mess up? Flag the unrelated incidents
よく似たインシデント
Did our AI mess up? Flag the unrelated incidents