CSETv0 分類法のクラス
分類法の詳細Problem Nature
Unknown/unclear
Physical System
Software only
Level of Autonomy
Low
Nature of End User
Amateur
Public Sector Deployment
No
Data Inputs
creatinine levels, age, sex, race
CSETv1 分類法のクラス
分類法の詳細Incident Number
79
AI Tangible Harm Level Notes
There is no AI. The harm comes from a formula that uses race as a factor.
Notes (special interest intangible harm)
4.1 - Black patients overlooked by the calculation because of built-in points had their access to critical public healthcare reduced.
Special Interest Intangible Harm
yes
Risk Subdomain
1.3. Unequal performance across groups
Risk Domain
- Discrimination and Toxicity
Entity
Human
Timing
Post-deployment
Intent
Unintentional
インシデントレポート
レポートタイムライン
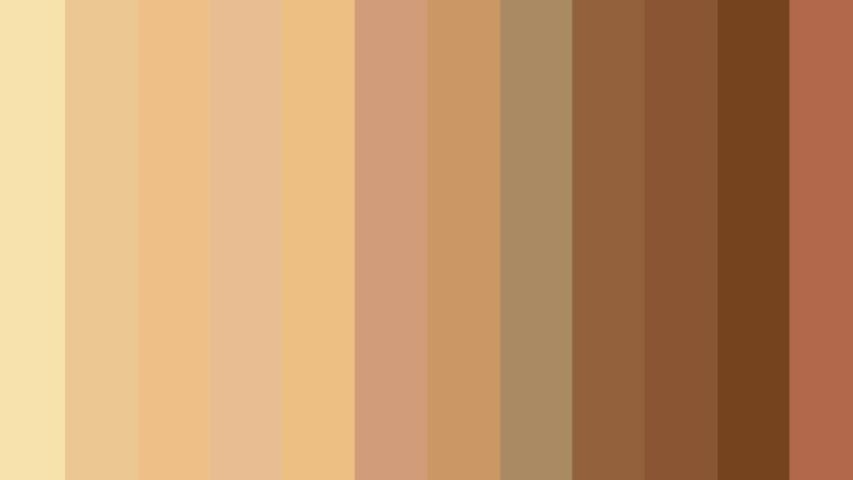
For years, physicians and medical students, many of them Black, have warned that the most widely used kidney test — the results of which are based on race — is racist and dangerously inaccurate. Their appeals are gaining new traction, with …

BACKGROUND: Advancing health equity entails reducing disparities in care. African-American patients with chronic kidney disease (CKD) have poorer outcomes, including dialysis access placement and transplantation. Estimated glomerular filtra…
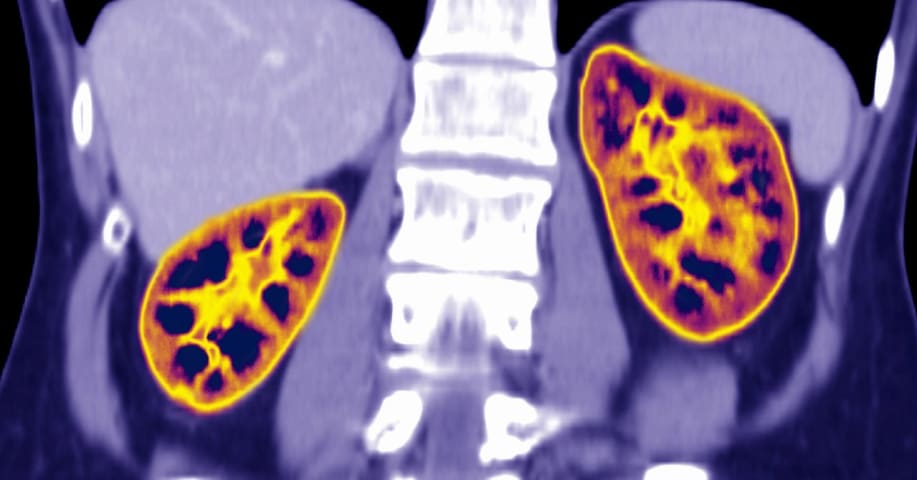
BLACK PEOPLE IN the US suffer more from chronic diseases and receive inferior health care relative to white people. Racially skewed math can make the problem worse.
Doctors often make life-changing decisions about patient care based on algo…