概要: In the “The Nature Conservancy Fisheries Monitoring” competition on the data science competition website Kaggle, a number of competitors overfit their image classifier models to a poorly representative validation data set.
推定: Individual Kaggle Competitorsが開発し提供したAIシステムで 、Individual Kaggle Competitorsに影響を与えた
CSETv0 分類法のクラス
分類法の詳細Problem Nature
Indicates which, if any, of the following types of AI failure describe the incident: "Specification," i.e. the system's behavior did not align with the true intentions of its designer, operator, etc; "Robustness," i.e. the system operated unsafely because of features or changes in its environment, or in the inputs the system received; "Assurance," i.e. the system could not be adequately monitored or controlled during operation.
Robustness
Physical System
Where relevant, indicates whether the AI system(s) was embedded into or tightly associated with specific types of hardware.
Software only
Level of Autonomy
The degree to which the AI system(s) functions independently from human intervention. "High" means there is no human involved in the system action execution; "Medium" means the system generates a decision and a human oversees the resulting action; "low" means the system generates decision-support output and a human makes a decision and executes an action.
Low
Nature of End User
"Expert" if users with special training or technical expertise were the ones meant to benefit from the AI system(s)’ operation; "Amateur" if the AI systems were primarily meant to benefit the general public or untrained users.
Expert
Public Sector Deployment
"Yes" if the AI system(s) involved in the accident were being used by the public sector or for the administration of public goods (for example, public transportation). "No" if the system(s) were being used in the private sector or for commercial purposes (for example, a ride-sharing company), on the other.
No
Data Inputs
A brief description of the data that the AI system(s) used or were trained on.
Images captured on fishing boats
CSETv1 分類法のクラス
分類法の詳細Incident Number
The number of the incident in the AI Incident Database.
61
Risk Subdomain
A further 23 subdomains create an accessible and understandable classification of hazards and harms associated with AI
7.3. Lack of capability or robustness
Risk Domain
The Domain Taxonomy of AI Risks classifies risks into seven AI risk domains: (1) Discrimination & toxicity, (2) Privacy & security, (3) Misinformation, (4) Malicious actors & misuse, (5) Human-computer interaction, (6) Socioeconomic & environmental harms, and (7) AI system safety, failures & limitations.
- AI system safety, failures, and limitations
Entity
Which, if any, entity is presented as the main cause of the risk
AI
Timing
The stage in the AI lifecycle at which the risk is presented as occurring
Post-deployment
Intent
Whether the risk is presented as occurring as an expected or unexpected outcome from pursuing a goal
Unintentional
インシデントレポート
レポートタイムライン
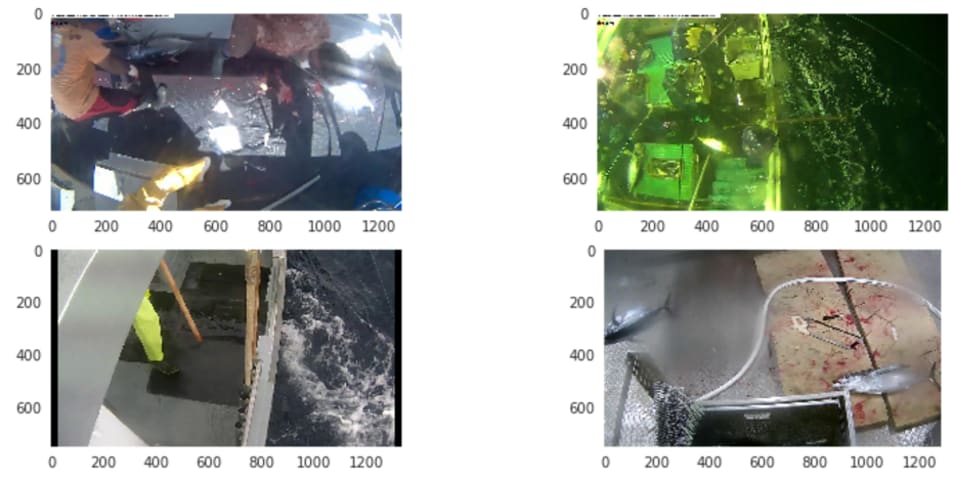
What I’ve learned from Kaggle’s fisheries competition
Gidi Shperber Blocked Unblock Follow Following May 1, 2017
TLDR:
Me and my Kaggle partner, have recently participated in “The Nature Conservancy Fisheries Monitoring” (hereby: “fisheries…
バリアント
「バリアント」は既存のAIインシデントと同じ原因要素を共有し、同様な被害を引き起こし、同じ知的システムを含んだインシデントです。バリアントは完全に独立したインシデントとしてインデックスするのではなく、データベースに最初に投稿された同様なインシデントの元にインシデントのバリエーションとして一覧します。インシデントデータベースの他の投稿タイプとは違い、バリアントではインシデントデータベース以外の根拠のレポートは要求されません。詳細についてはこの研究論文を参照してください
よく似たインシデント
Did our AI mess up? Flag the unrelated incidents
よく似たインシデント
Did our AI mess up? Flag the unrelated incidents