インシデントのステータス
CSETv0 分類法のクラス
分類法の詳細Problem Nature
Specification
Physical System
Software only
Level of Autonomy
Medium
Nature of End User
Amateur
Public Sector Deployment
No
Data Inputs
User entered translation requests
CSETv1 分類法のクラス
分類法の詳細Incident Number
59
AI Tangible Harm Level Notes
Although AI was implicated in the adverse outcome, this incident has no tangible harm.
Notes (special interest intangible harm)
The study found biases related to gender and age in Google Translate. Additional biases have been found in Natural Language Processing in general.
Special Interest Intangible Harm
yes
CSETv1_Annotator-1 分類法のクラス
分類法の詳細Incident Number
59
AI Tangible Harm Level Notes
Although AI was implicated in the adverse outcome, there is no tangible harm in this incident.
Special Interest Intangible Harm
yes
Date of Incident Year
2016
Estimated Date
Yes
Multiple AI Interaction
no
CSETv1_Annotator-3 分類法のクラス
分類法の詳細Incident Number
59
Notes (special interest intangible harm)
The study found biases related to race, gender, age, and sentiment in the AI systems
Special Interest Intangible Harm
yes
Notes (AI special interest intangible harm)
The study found biases related to race, gender, age, and sentiment in the AI systems' understanding of words.
Date of Incident Year
2017
Date of Incident Month
5
インシデントレポート
レポートタイムライン
- 情報源として元のレポートを表示
- インターネットアーカイブでレポートを表示
Artificial intelligence and machine learning are in a period of astounding growth. However, there are concerns that these technologies may be used, either with or without intention, to perpetuate the prejudice and unfairness that unfortunat…

- 情報源として元のレポートを表示
- インターネットアーカイブでレポートを表示
Even artificial intelligence can acquire biases against race and gender
One of the great promises of artificial intelligence (AI) is a world free of petty human biases. Hiring by algorithm would give men and women an equal chance at work, t…
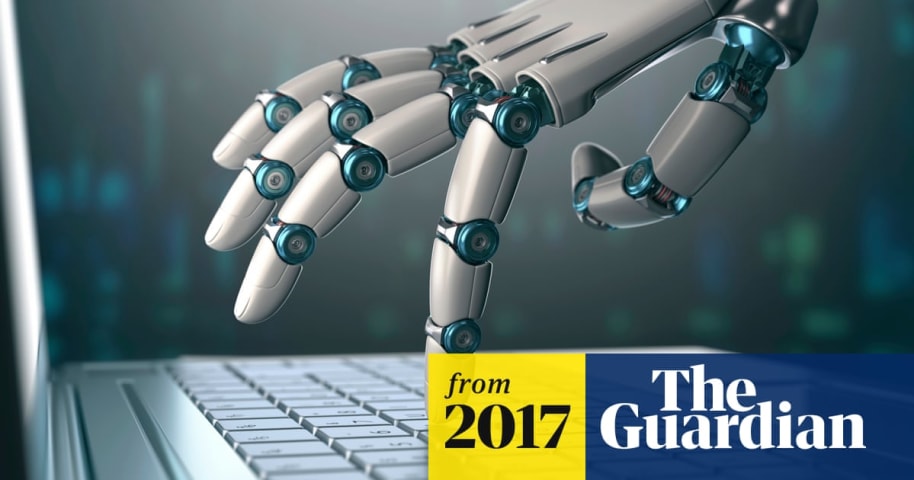
- 情報源として元のレポートを表示
- インターネットアーカイブでレポートを表示
Machine learning algorithms are picking up deeply ingrained race and gender prejudices concealed within the patterns of language use, scientists say
An artificial intelligence tool that has revolutionised the ability of computers to interpr…
- 情報源として元のレポートを表示
- インターネットアーカイブでレポートを表示
In debates over the future of artificial intelligence, many experts think of these machine-based systems as coldly logical and objectively rational. But in a new study, Princeton University-based researchers have demonstrated how machines c…
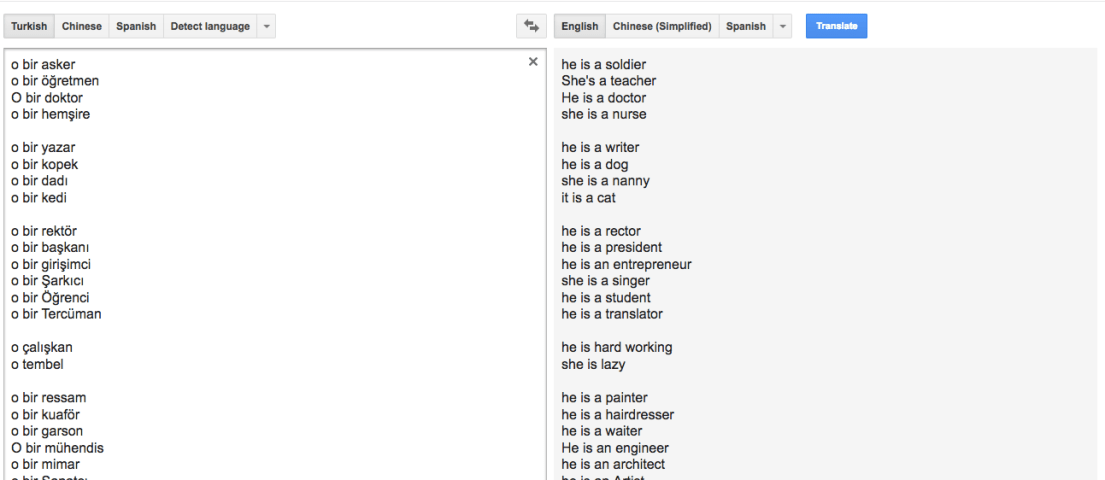
- 情報源として元のレポートを表示
- インターネットアーカイブでレポートを表示
In the Turkish language, there is one pronoun, “o,” that covers every kind of singular third person. Whether it’s a he, a she, or an it, it’s an “o.” That’s not the case in English. So when Google Translate goes from Turkish to English, it …
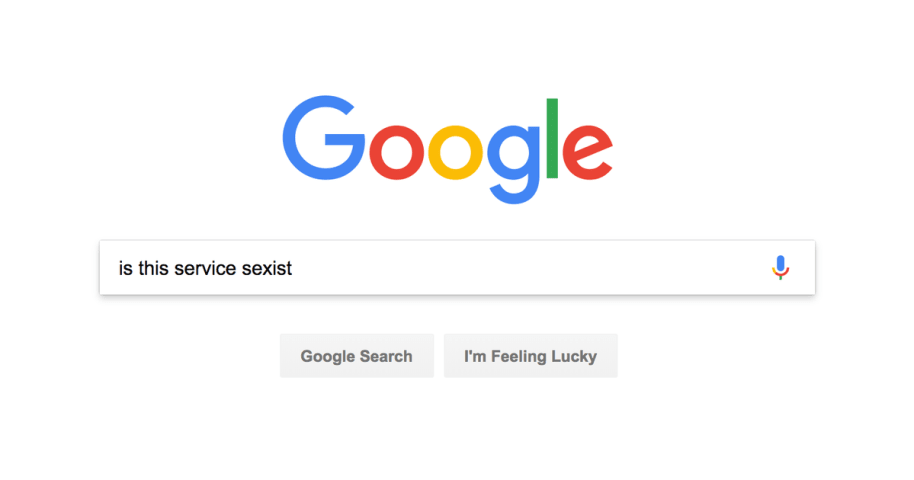
- 情報源として元のレポートを表示
- インターネットアーカイブでレポートを表示
So much of our life is determined by algorithms. From what you see on your Facebook News Feed, to the books and knickknacks recommended to you by Amazon, to the disturbing videos YouTube shows to your children, our attention is systematical…
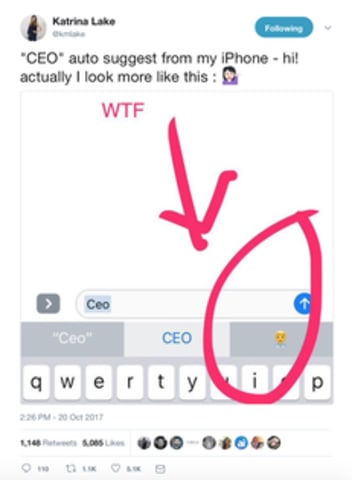
- 情報源として元のレポートを表示
- インターネットアーカイブでレポートを表示
Image via Twitter
Parents know one particular challenge of raising kids all too well: teaching them to do what we say, not what we do.
A similar challenge has hit artificial intelligence.
As more apps and software use AI to automate tasks, …
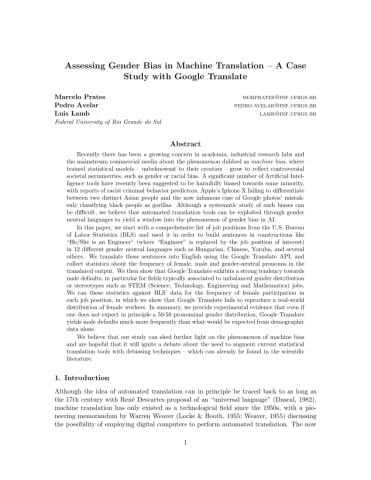
- 情報源として元のレポートを表示
- インターネットアーカイブでレポートを表示
Recently there has been a growing concern about machine bias, where trained statistical models grow to reflect controversial societal asymmetries, such as gender or racial bias. A significant number of AI tools have recently been suggested …
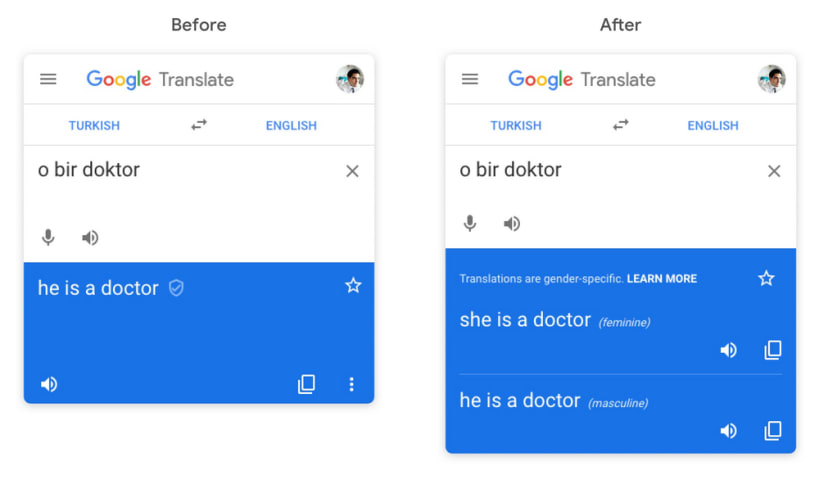
- 情報源として元のレポートを表示
- インターネットアーカイブでレポートを表示
Google is making an effort to reduce perceived gender bias in Google Translate, it announced today. Starting this week, users who translate words and phrases in supported languages will get both feminine and masculine translations; “o bir d…

- 情報源として元のレポートを表示
- インターネットアーカイブでレポートを表示
An experiment shows that Google Translate systematically changes the gender of translations when they do not fit with stereotypes. It is all because of English, Google says.
If you were to read a story about male and female historians trans…
バリアント
よく似たインシデント
Did our AI mess up? Flag the unrelated incidents
よく似たインシデント
Did our AI mess up? Flag the unrelated incidents