インシデントのステータス
インシデントレポート
レポートタイムラ イン
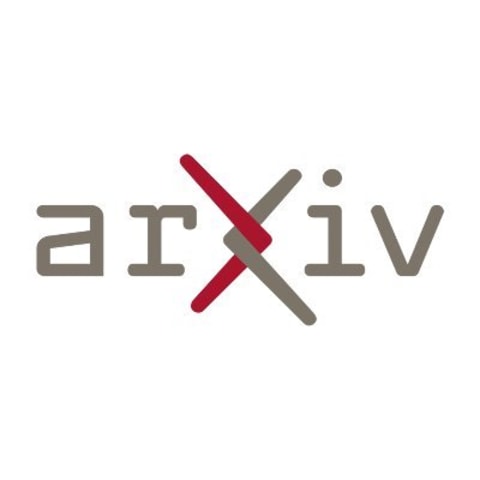
- 情報源として元のレポートを表示
- インターネットアーカイブでレポートを表示
The enormous financial success of online advertising platforms is partially due to the precise targeting features they offer. Although researchers and journalists have found many ways that advertisers can target---or exclude---particular gr…
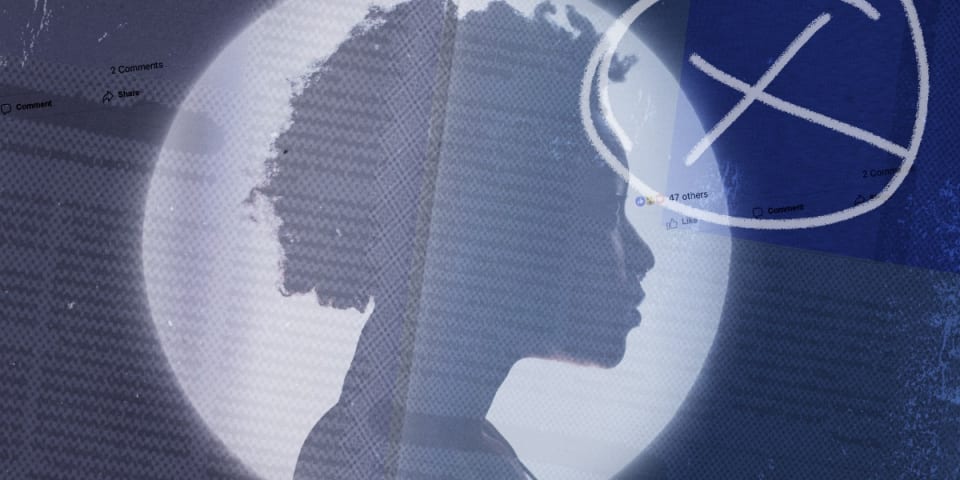
- 情報源として元のレポートを表示
- インターネットアーカイブでレポートを表示
Facebook is withholding certain job ads from women because of their gender, according to the latest audit of its ad service.
The audit, conducted by independent researchers at the University of Southern California (USC), reveals that Facebo…
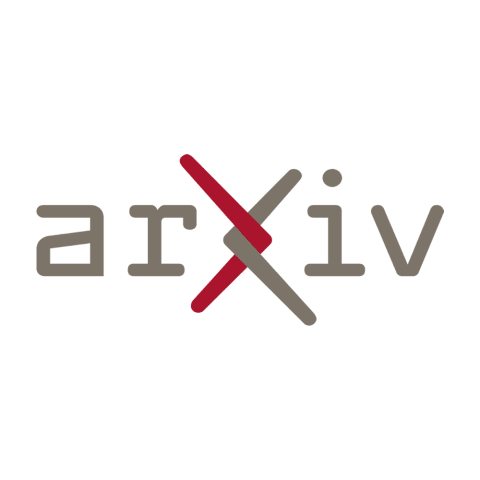
- 情報源として元のレポートを表示
- インターネットアーカイブでレポートを表示
Ad platforms such as Facebook, Google and LinkedIn promise value for advertisers through their targeted advertising. However, multiple studies have shown that ad delivery on such platforms can be skewed by gender or race due to hidden algor…
バリアント
よく似たインシデント
Did our AI mess up? Flag the unrelated incidents
よく似たインシデント
Did our AI mess up? Flag the unrelated incidents